Executive Summary
The present system of data and information management in the Reserve Bank evolved over several years in response to the emerging needs of the Reserve Bank and to disseminate information as a ‘public good’. With increasing integration with the global economy and the growing complexity of the economic structure, the information needs have increased considerably and data gaps are being experienced in various domains of central banking. To address the concerns about data gaps and to place the information management on a more technologically mature footing, the Committee on Data and Information Management set up by the Reserve Bank reviewed the current system of data collection, dissemination and management processes and examined the feasibility of moving towards granular, multi-purpose data collection and more integrated and structured data production processes. The Committee makes the following key recommendations:
-
To address major data gaps in monetary policy-making, the Committee recommends the compilation of various indictors, such as producer price index, services sector output and price index, labour force survey, urban wages, retail sales, construction activity survey, and surveys of household indebtedness through co-ordination with the relevant government agencies.
-
The scope and coverage of the financial soundness indicators may be expanded to non-bank segments of the financial sector and the frequency of compiling sectoral flow of funds may be made quarterly starting with the financial sector.
-
The envelope of data elements across various returns in different information domains should be rationalised and the data should be moved to an enterprise-wide data warehouse (EDW) with appropriate access rights for users.
-
For primary data, the Committee recommends that it would be optimal to dissolve the returns into data elements, which can be organised with relevant attributes and dimensions in the form of logically coherent datasets. This would also serve the current purpose of returns.
-
The Committee suggests that the values of various attributes and dimensions may be standardised to enable collation of data from different domains.
-
XBRL (eXtensible Business Reporting Language), is a XML-based non-proprietary open standard that is used to prepare, exchange and publish business information. The Committee recommends the use of XBRL as the principal vehicle for data submission.
-
The data for which XBRL taxonomies and validation rules have been made available should be submitted through XBRL instance documents generated directly from the central repositories of the banks without any manual intervention.
-
Using the secure network connections between the RBI server and the banks’ ADF servers, the contents of the dataset may be pulled or pushed and loaded onto the RBI’s server in an automated manner.
-
For granular micro (account/lowest unit-level data) and transactional multi-dimensional data, the Reserve Bank should develop and provide specific details of RDBMS/text file structures along with standardised code lists and basic validation rules.
-
Big-data architecture may be considered for micro and transactional datasets considering their high volume, velocity and multi-dimensional nature.
-
The Committee recommends that common systems may be used for data collection, storage and compilation in the Bank. A unified approach across various domains provides an opportunity to procure and deploy professional-grade tools for data quality management as part of the data warehouse infrastructure.
-
A dissemination policy for the Bank may be formulated and used as a guiding principle for disseminating or sharing information with various classes of users, within and outside the Bank.
-
A review of the coverage of the statistical publications may be undertaken internally by the Reserve Bank to streamline the process and to remove/reduce overlaps.
-
The Committee recommends that it will be convenient to separate press releases that focus on specific data from the other press releases, which should be clubbed under the separate head of ‘Statistical Releases’ for easier access to historical data.
-
To enhance the data governance framework, the Bank should identify and assign the role of Chief Information Officer (CIO) to a senior official and the entire data requirements of the Reserve Bank should be managed centrally for coherence, consistency and accountability.
-
The Committee suggests that function of information management may be organised under an independent Information Management Unit (IMU) carved out of the current Department of Statistics and Information Management (DSIM) and manned by statisticians and IT experts, with support from officials with the requisite domain knowledge.
Chapter 1
Introduction
1.1 The Reserve Bank of India (RBI), which performs multiple functions ranging from monetary policy to regulation and supervision, requires a large variety of information to effectively discharge its responsibilities. The present system of collecting, managing and utilising information evolved over several years in response to the emerging needs of the Reserve Bank and with the objective of disseminating information as a ‘public good’. With increasing integration with the global economy and the growing complexity of the economic structure, the information needs have expanded considerably. Therefore, gaps are being experienced in various domains of information required for policy formulation as also in efficient management of information.
1.2 To address these concerns about data gaps and to place information management on a more technologically mature footing, the Reserve Bank constituted a Committee to comprehensively review the current system of data collection, dissemination and management and to examine the feasibility of moving towards granular, multi-purpose data collection and more integrated and structured data production processes.
1.3 The terms of reference of the Committee were:
-
To review the availability, coverage, frequency, timeliness and quality of the existing data/surveys/information used for: (a) monetary policy, (b) macro- financial policy and (c) supervision.
-
To review the recommendations of earlier Committees/Working Groups on data gaps and information management in the Reserve Bank.
-
In the light of (i) and (ii) above, suggest changes in the process of receipt, storage, access, management and dissemination of monetary, macro-financial and supervisory data.
-
In order to achieve (iii) above, suggest necessary institutional and technological changes within the Reserve Bank to make available consistent data from a single source for all user groups as well as for management decision-making.
-
To suggest complementary technological and process changes at the level of banks so that granular data are made available from the source system that could ensure data integrity.
- To consider any other related matters.
1.4 The composition of the Committee was as follows:
(i) |
Shri Deepak Mohanty, Executive Director, RBI |
Chairman |
(ii) |
Prof. Bimal Roy, Director, Indian Statistical Institute |
Member |
(iii) |
Prof. Pami Dua, Head, Delhi School of Economics |
Member |
(iv) |
Dr. Susan Thomas, Assistant Professor, Indira Gandhi Institute of Development Research |
Member |
(v) |
Prof. N. L. Sarda, Indian Institute of Technology, Mumbai |
Member |
(vi) |
Shri Kajal Ghosh, Chief General Manager, State Bank of India |
Member |
(vii) |
Shri Anil Jaggia, Chief Information Officer, HDFC Bank |
Member |
(viii) |
Shri Chandan Sinha, Principal Chief General Manager, Department of Banking Operations and Development, RBI@ |
Member |
(ix) |
Shri P. R. Ravi Mohan, Chief General Manager-in-Charge, Department of Banking Supervision, RBI @@ |
Member |
(x) |
Shri A. K. Bera, Principal Chief General Manager, Urban Banks Department, RBI |
Member |
(xi) |
Dr. A. S. Ramasastri, Chief General Manager–in-Charge, Department of Information Technology, RBI@@@ |
Member |
(xii) |
Dr. B. K. Bhoi, Adviser, Monetary Policy Department, RBI |
Member |
(xiii) |
Shri Sanjay Hansda, Director, Department of
Economic and Policy Research, RBI |
Member |
(xiv) |
Dr. Abhiman Das, Director, Department of Statistics and Information Management, RBI |
Member |
(xv) |
Dr. A. K. Srimany, Adviser, Department of
Statistics and Information Management, RBI * |
Member-Secretary |
@At present, Executive Director
@@ On taking charge of DBS before the first meeting of the Committee, replaced Shri P. Vijaykumar as mentioned in the original memorandum
@@@ At present, Director, IDRBT, Hyderabad
* At present, Officer-in-Charge, DSIM |
1.5 The Department of Statistics and Information Management (DSIM) provided secretarial support to the Committee. The members of the secretariat consisted of Dr. A. R. Joshi, Shri Anujit Mitra, Shri Ravi Shankar, Shri N. Senthil Kumar, Dr. Y. K. Gupta, Dr. Pradip Bhuyan, Shri Shiv Shankar and Shri Amarnath Yadav.
1.6 The Committee also consulted experts from academia, banks, technology providers and other central banks, particularly the European Central Bank, through different channels. The Committee organised its deliberations through meetings of the full committee, sub-groups and other formal and informal consultations. Dr. Raghuram G. Rajan, Governor, addressed the first meeting of the Committee to lay out the background and to provide an overall vision. The composition of sub-groups and their terms of reference are provided in Annex 1.
Chapter 2
Data Gaps
Introduction
2.1 The Committee recognised that the information system is spread across different functional areas and it will not be practical to identify each and every data element. It, therefore, decided to prioritise its activities and focus where data gaps and inconsistency are being felt more severely due to overlap of various information systems. Accordingly, the Committee identified three generic information domains that require focused attention: (i) data related to monetary and financial stability (ii) data on financial inclusion and (iii) micro prudential supervisory data.
2.2 The main vehicle for gathering information from regulated entities is the 'returns' submitted by these entities in the prescribed format and periodicity. Out of around 220 returns submitted by Scheduled Commercial Banks excluding Regional Rural Banks (referred to as SCBs henceforth), the returns submitted to the Department of Banking Supervision (DBS), Department of Banking Operations and Development (DBOD), Department of Statistics & Information Management (DSIM) and Monetary Policy Department (MPD) cover most of the data elements reported by the banks. The Committee noted earlier attempts to rationalise the returns. The major thrust in this direction came from initiatives to move the reporting to the Online Report Filing System (ORFS), using eXtensible Business Reporting Language (XBRL) and Automated Data Flow (ADF).
2.3 The Committee noted that the Reserve Bank has set up a data warehouse (DW) that has become an important data communication channel of the Bank. The data items in the DW include both data compiled internally by the Reserve Bank and data sourced from other organisations such as the Central Statistics Office (CSO). The Committee reviewed the sector-wise details of data items available in the DW. The sectors covered in the DW are agriculture, industry, national income, prices, banking statistics, monetary statistics, payment system statistics and financial markets. An increasing number of statistical publications are generated from the DW, but the bulk of the available data, particularly supervisory statistics, does not reside in the DW. Within the DW there is also some duplication in macro-financial statistics between what is available in the Handbook of Statistics on Indian Economy and what is available in the Database on Indian Economy. The Committee recommends that the DW should be converted into an enterprise-wide data warehouse (EDW) where all the key data, including supervisory data, should reside without duplication.
Monetary policy
2.4 The Committee noted that the database for monetary policy formulation has improved over time. The available information set has been augmented by several forward-looking surveys. Recently, an all-India consumer price index was released, but it has significant data gaps. With regard to price statistics and output indicators, the Committee recommends the compilation of a producer’s price index, a services output indicator and a services price index. The database may also be augmented to cover agricultural commodity futures prices. In addition, monitoring of price and other developments at regional level, which may be relevant for the monetary policy formulation, needs to be strengthened.
2.5 Another major data gap relates to the timely availability of information on employment and wages for monetary policy formulation. The Committee reviewed international experiences and found that the majority of countries collect employment/ unemployment statistics on monthly/quarterly basis. Following these best practices, the Committee recommends instituting a quarterly ‘labour force survey’ in India to provide internationally comparable employment/unemployment statistics. This issue may be taken up with the Government, and the Reserve Bank should extend the necessary support.
2.6 Although data on rural wages are available from the Labour Bureau, data are not available for urban wages. The Reserve Bank, along with the Government, should explore the possibility of collecting data on urban wages. In the interim, the Reserve Bank may launch a quarterly sample survey to collect data on urban wages across broad industrial groups.
2.7 There is a data gap in construction sector activity. Typical measures for real estate construction activity could include a housing start-up index (HSUI), land prices index and construction-cost index. The HSUI is considered a major economic activity indicator. The Committee notes that some work has been done in collaboration with the Ministry of Housing towards the HSUI and recommends that the Reserve Bank may construct and release an HSUI at a quarterly frequency.
2.8 The Working Group on Surveys, 2009 (Chairman: Deepak Mohanty) reviewed and recommended the need for new surveys to fill information gaps. Several surveys that were recommended by the Working Group have been introduced to provide forward-looking information on the economic outlook. However, two gaps remain: (i) a survey on the outlook for the services sector and (ii) a survey on household indebtedness. In addition, there is a data gap in assessing the consumption demand for the economy. Towards this, several central banks and national statistics offices conduct retail sales surveys. The Committee recommends that the Reserve Bank may initiate work on these surveys. While a household indebtedness survey may be conducted annually, the other two surveys should be undertaken every quarter.
Financial stability
2.9 In the context of the G-20 data gap initiative (DGI), while the Reserve Bank reports data on 12 core and 13 encouraged financial soundness indicators (FSI) for banks, these indicators do not cover non-bank financial companies (NBFCs) and other financial corporations such as insurance, pensions and other asset management sectors. The Committee recommends that the scope and coverage of the FSI should be expanded to other segments of the financial sector.
2.10 India is currently compliant with the IMF Special Data Dissemination Standard (SDDS). However, SDDS Plus requires a minimum set of internationally comparable sectoral financial balance sheets with a set of prescribed sub-sectors of the financial corporations. The Committee recommends that efforts should be made to compile quarterly flow-of-funds accounts starting with the financial sector.
2.11 There also appears to be a lack of adequate information about the securities markets. For example, there is a gap in terms of finance raised through either private placements or through public issues. Credit derivative markets have been a critical input for capturing stress in the real sector. While these markets are underdeveloped in India, instruments such as credit default swaps (CDS) on Indian firms are available off-shore. Such information should be collected and maintained within the data and information management systems at the Reserve Bank as an input into assessing financial stability. Further, the scope and coverage of data on unhedged forex exposure of corporates, which are important from the point of view of external sector stability, need to be strengthened.
Financial inclusion
2.12 In India, the main objective of financial inclusion is to ensure access to a range of financial services that includes savings, credit, insurance, remittance and other banking/ payment services to all ‘bankable’ households and enterprises at a reasonable cost. Recently, the Nachiket Mor Committee on Comprehensive Financial Services for Small Businesses and Low Income Households recommended that supply-side data elements of financial inclusion may be taken into account while preparing the data elements for banks and NBFCs.
2.13 The Master Office File (MOF) and Basic Statistical Returns (BSR) are the most reliable sources of supply-side financial inclusion data in India. While the MOF provides bank branch statistics, the BSR provides granular data on the deposit and credit of Scheduled Commercial Banks and Regional Rural Banks. Additionally, the Rural Planning and Credit Department (RPCD) collects data pertaining to 46 items on financial inclusion from RRBs. Along with data on the volume of bank/branch/business correspondent (BC) outlets, the Committee recommends that the possibility of including Urban Co-operative Banks (UCBs) under the MOF may be explored. Further, MOF-like structure for NBFCs may also be designed to maintain branch information on NBFCs.
2.14 While the major part of supply-side data can be captured through banking statistics, there is lack of reliable demand-side data on financial inclusion. The Committee recommends instituting a bi-annual survey to provide requisite data on measuring access, quality, usage and welfare impact of various financial services and products.
Micro-prudential data
Commercial banks
2.15 The data/information required for supervision can be classified into two groups based on its source, viz. (i) submitted by banks, (ii) generated/compiled by the supervisor. Further, the form of these data can be classified as either structured (e.g., numeric/textual) or unstructured (e.g., documents/files). Examples of various data/information types used for supervision are given in Table 1.
Table 1: Type of Information Used for Banking Supervision |
Data Type |
Submitted by Bank |
Generated/Compiled by Supervisor |
Structured |
Numerical/
Financial |
1) DSB Returns (XBRL)
2) Fraud Returns
3) FID Returns
4) RBS Risk data (Data Collector application)
5) Financial Conglomerate Return (Excel)
6) Ad hoc data (Data Collector application) |
7) Standard Annexes as part of onsite inspection
8) Assessment of key financials/capital including validation/re-assessment of RBS risk data furnished by bank
9) Scores for aggregations of various risks as part of IRISc model
10) Thematic/Sector/Industry/other bank-wide studies |
Textual |
11) RBS Control gap information (Data Collector application)
12)
RBS Compliance information (Data Collector application) |
13) Comments/additional information on Control gap and Compliance by SSM
14) Comments by Quality Assurance Division |
Unstructured |
|
15) Annual Reports
16)
Policy Documents
17)
Board Minutes
18) Reports of External Auditors |
19) Working documents for supervisory assessment
20)
Supervisory Reports
21)
BFS Reports
22) Communications to banks |
2.16 For effective rollout of Risk-Based Supervision (RBS), the MIS solution must be effective, user-friendly and capable of seamlessly generating two important sets of collated information: (i) Risk Profile of banks (risk-related data – mostly new data elements), and (ii) Bank Profile (mostly financial data – DSB Returns and additional granular data). The Committee recommends that the envelope of data elements across returns should be rationalised and the supervisory data should be moved to the enterprise-wide data warehouse (EDW) with appropriate access rights for users. The unstructured and interactive components of the RBS data may be maintained separately, with appropriate links with the EDW.
Non-banking financial companies
2.17 Under the provisions of the RBI Act, the regulation and supervision of Non-Banking Financial Companies (NBFCs) is the responsibility of the Reserve Bank. NBFCs are classified into two categories - deposit taking (NBFCs-D) and non-deposit taking (NBFCs-ND) - depending on whether or not they accept public deposits. The latter group of companies with assets of ` 1 billion and above is classified as NBFCs-ND-SI (Systemically Important1).
2.18 Regulatory prescriptions are different for all these categories of NBFCs. Capital adequacy requirements, liquid assets maintenance, exposure norms and ALM discipline apply to NBFCs-D companies. NBFCs-ND-SI companies are subject to prudential regulations such as capital adequacy requirements and exposure norms along with reporting requirements. Other NBFCs-ND-SI companies are subject to minimal regulation2.
2.19 NBFCs that have assets of ` 1 billion and above but do not accept public deposits are required to submit the following returns: (i) quarterly statement of capital funds, risk weighted assets, risk asset ratio etc., for the company – NBS 7 (ii) monthly Return on Important Financial Parameters of the company (iii) ALM Statement on short-term dynamic liquidity [NBS-ALM1] (monthly) (v) ALM Statement of structural liquidity [NBS-ALM2] (half yearly) and (vi) ALM statement of interest rate sensitivity [NBS-ALM3] (half yearly).
2.20 During the discussion, it was observed that 10 per cent of the companies accounted for around 90 per cent of the total assets of the NBFC sector. A perusal of the returns submitted by NBFCs revealed that there is significant duplication in the information provided through various returns. The Committee recommends that these returns may be rationalised by identifying major data elements. A sub-group constituted by the Committee has already identified a number of elements that should form the basis for rationalisation. The Committee recommends an online data collection mechanism for larger NBFCs.
Urban co-operative banks
2.21 The regulation and supervision of Urban Co-operative Banks (UCBs) is vested with the Reserve Bank in respect of their banking activities. UCBs are primarily classified as scheduled or non-scheduled. As at the end of March 2013, there were 1606 UCBs of which 51 were scheduled and accounted for 45 per cent of the total assets of UCBs. The scheduled SCBs filed around 40 returns, and a scrutiny found considerable overlap of data elements in the returns. The Committee recommends that these returns should be rationalised by identifying major data elements. A sub-group constituted by the Committee has already identified a number of elements that should form the basis for rationalisation.
2.22 In the UCB sector, 84 per cent of the assets are held by Tier II UCBs, accounting for only 26 per cent of the total number of UCBs. The Committee recommends introducing an online data collection mechanism for Tier II UCBs.
Chapter 3
Data Model for Information System
Current data model
3.1 The Committee noted that the existing data compilation systems give priority to departmental information systems, which are the primary feeders of the management information system. This has limited the scope for an enterprise-wide data model to emerge. The Committee recommends that the Reserve Bank should move to an overall high-level information model that is derived from the information needs of the various arms of the Bank. The availability of sufficiently mature technology solutions and experienced manpower in the Bank should ensure that the high-level model can be translated into an information system that provides necessary information to users as per their needs.
3.2 As a first step in this direction, the Committee noted that there is a need to define the data required by the Reserve Bank in its entirety so that the regulated entities, which submit the information, can also organise their systems in line with the reporting requirements specified by the Bank. The Committee felt that for primary data it would be optimal to dissolve the returns into data elements; these can be organised into datasets that contain logically coherent data elements. For the datasets, which are secondary in nature, the input data structure is to be taken as available.
3.3 The Committee considered the possibility of getting all the information in granular form. After considerable deliberation, it concluded that some data are relevant only at the aggregate level. Thus, the focus should be on collecting relevant, coherent and unduplicated data from banks. This will also reduce the reporting burden on banks. The Committee, therefore, recommends three generic data collection structures: (i) aggregated data, (ii) distributed aggregates, and (iii) granular data depending on the relevance of data at different operational hierarchies.
Structure of returns
3.4 Currently, most of the returns are organised as a set of sheets to collect logically similar data in terms of structure or subject area. An examination and grouping of the contents of the majority of input forms reveal the following types of components:
-
Measures – variables that take numerical values and are amenable to mathematical operations. For example, amount outstanding, credit limit and number of accounts.
-
Concepts – are labels at a broad level, which have several manifestations. For example, deposits, credit and capital.
-
Elements – are labels that are most clearly defined and cannot be broken up further. For example, paid-up capital at the bank level and savings deposits at the branch level.
-
Attributes – are possible features of the variables/ elements, such as audited/ unaudited and provisional/revised.
-
Distributions – are characteristics of the measures for which break-up against identified lists is required. For example, sectoral deployment of credit and maturity profile of deposits.
-
Dimensions– are similar to distributions but are not necessarily mutually exclusive and exhaustive - for example, deposits and its specified subsets such as ‘from banks in India’.
All the components, except the measures, are normally hard-coded in the return formats.
3.5 The present returns can be classified under three major structures. In the first case, the returns contain a hierarchical list (D1) of elements with a parent-child relationship. For example, in the balance sheet analysis (BSA) return, various elements in the banks’ financial statement are presented in hierarchical form. Elements for which data are to be collected are presented row-wise, while related measures such as amount outstanding, number of accounts, outflow and inflow are presented in columns. Some measures among the various elements may be linked through arithmetic relationships. In the second case, certain aspects are connected with a distribution (D2). For example, in the return on asset quality (RAQ), the assets are classified by type of asset and sector. The third case is a multi-dimensional granular list (D3), with columns of numeric and non-numeric types. For example, the Basic Statistical Return 1 (BSR1) is used to collect account-level information on bank credit with various characteristics, such as sector, interest rate and type of borrower. In addition to these three types, there is a fourth type that may be labelled D*. This is a list of non-standard elements/transactions, such as a list of offices and directors.
Suggested data model
3.6 The Committee analysed the merits and demerits of the existing models of data collection and felt that the data collection process would be more efficient if it was re-oriented towards an element-based, simplified and standardised process under a generic model structure that was suitable for both primary and secondary data. The Committee also suggests that the values of various attributes and dimensions should be standardised to enable the collation of data from different domains. A simplified representation of the proposed generic models for datasets (GMs) is presented in Table 2.
Metadata directory
3.7 An efficient and effective data management function in the Reserve Bank means that the Bank should be in a position to know at any time point what data are being collected from various constituencies at what intervals and in what form. The Committee recommends that it will be useful to prepare a Data Element Directory (proposed structure given in Annex 2) that lists data elements with their related attributes to be collected from various constituencies.
3.8 A Data Dissemination Directory with details of variables, definitions, statistical attributes and other time series attributes needs to be prepared and maintained as part of the metadata directory. This will also facilitate dissemination of time series data using the Statistical Data and Metadata Exchange (SDMX) standard. A Data Channel Directory with details of data channels, data sources and mode of transfer may need to be maintained for monitoring and follow-up.
3.9 The data element, data dissemination and data channel directories should be maintained centrally and in a proper database format so that they can be accessed by data managers, data users and software developers. These directories will form the backbone of data management processes such as collection, storage and dissemination.
Chapter 4
Technology and Design
Introduction
4.1 Traditional constraints on capturing, transmitting, storing and processing large amounts of data have now been overcome with technological progress. The Reserve Bank can therefore afford to liberally collect and process large amounts of micro and transactional data by setting up appropriate technological capabilities and processes that, in turn, can generate a continuous flow of information for the Bank’s regulatory, supervisory and policy-making purposes.
4.2 The various constituencies that the Bank deals with are Scheduled Commercial Banks (89), RRBs and State, Central, District Co-operative Banks (466), Urban Co-operative Banks (1,600), Primary Dealers (21) and Non-Banking Financial Companies (11,000)3. The collection and storage structures identified for the three generic models of datasets, as recommended in Chapter 3, viz., bank-level summarised measures (GM1), small generic distribution (GM2) and large specific multi-dimensional micro transactional/account's list (GM3), could serve as the backbone for the proposed architecture.
4.3 The design of the technical architecture proposed in this chapter is based on the following principles: simplicity, use of the existing infrastructure, scalability in terms of increased number and volume of reporting, gradual adoption, feasibility and the use of a layer of outsourced vendor personnel at various levels of statistics processes to reduce the operational burden and system complexity.
Technical architecture
4.4 The recommended architecture for data management in the Bank is illustrated in Chart 1.
System software requirements at the Reserve Bank
4.5 Some of the requirements for system software are already available in the Reserve Bank as part of the technical infrastructure: ETL Tool (Informatica), SFTP, Business Intelligence for OLAP (Business Object), Dashboard Tools and Oracle for the RDBMS.
4.6 Other system software needs to be acquired: (i) portal for Micro and SDMX-based macro data dissemination and for managing meta-data directory, data flow channels and code lists, (ii) database for managing large micro datasets and (iii) data quality tools.
Data collection mechanism
4.7 With the implementation of Phase I of the Automated Data Flow (ADF) project, most banks report that they are in a position to generate all returns to be submitted to the Reserve Bank from a single Central Repository (ADF server). Therefore, technical infrastructure, particularly ADF Central Repositories established at the banks’ end, is required for automated data flow between the banks and the Reserve Bank. The Reserve Bank’s Approach Paper on ADF (RBI, 2010) envisaged that banks would prepare a central repository that would contain all the data elements required for reporting to the Reserve Bank. Banks that have followed this vision in developing their central repositories will find it easier to migrate to the element-based data reporting envisaged by the Committee. Banks that have followed a return-based approach, probably as a measure of expediency, may have to make changes to their systems.
4.8 Bank-level aggregates, for which XBRL taxonomies and validation rules (XSD files) have been made available, should be submitted through XBRL instance documents generated directly from the central repositories of the banks without any manual interventions. The banks should validate the generated instance documents based on the XBRL taxonomy and validation rules before sending them to the Reserve Bank. Wherever automated generation of instant document is not feasible, data collection instruments in XBRL Excel templates may be used as an interim arrangement.
4.9 For granular micro (account/lowest unit-level data) and transactional multi-dimensional data, the Reserve Bank should develop and provide specific details of RDBMS/text file structures along with standardised code lists and basic validation rules so that banks can run the validation logics to ascertain that the datasets are submission-ready. Banks should also update a flag when they are ready for the Reserve Bank to pull their data. Using the secure network connections between the RBI server and the bank’s ADF server, the contents of the dataset will be pulled (ETL) or pushed (SFTP) and loaded onto the RBI server automatically as per the periodicity without any manual intervention (the ETL tool should check the flag before commencing extraction). An acknowledgement or the result of the loading process will be automatically communicated to the bank’s ADF co-ordination group for action, if necessary.
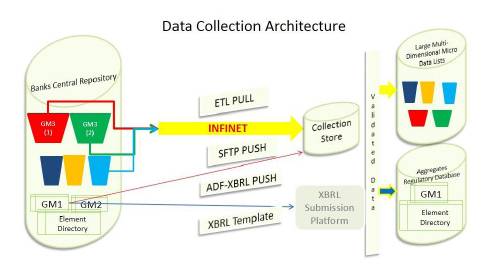
4.10 In the case of data elements under Generic Model GM2, in the majority of cases distributions can be directly generated based on the respective micro/transactional datasets (i.e., elements under Generic Model GM3). Until the elements under Generic Model GM3 are available, the proposed collection of elements under Generic Model GM2 will have to be used.
4.11 In the case of data elements under Generic Model GM3, static attributes that identify an entity and related transactional aspects, which continue to change over time, may be maintained separately. This arrangement will significantly reduce the volume of data flow, since the static portions need to be transferred to the Reserve Bank only in the case of new/ updated entities.
4.12 An effective Data Flow Monitoring System (DFMS) should be developed based on the Data Channel Directory to monitor data channels that connect various internal and external data sources and to ensure timely data flow in these channels through automatic or manual follow-up processes.
Data storage and processing
4.13 While the traditional RDBMS infrastructure in place in the Bank may be used for storage and retrieval of aggregated and finalised data, Big-data architecture may be considered for micro and transactional datasets given their high volume, velocity and multi-dimensional nature. Open source Big-data tools may also be considered.
4.14 To store raw data received directly from banks’ ADF servers, a separate collection store may be set up. The collection store will serve as a landing platform for all datasets directly pulled/pushed from banks’ ADF servers. This collection store will used be for datasets that are not submitted through the XBRL submission platform. The collection store will feed to the EDW staging layer. Data processing for statistical production such as data quality analysis, substitution and editing will be carried out at this stage.
4.15 Since a significant number of tasks related to data movement (between banks and the Reserve Bank) and validation are based on the ETL (Extract Transform Load) platform, the existing ETL platform may need to be strengthened so that it is able to connect different types of data sources and can extract data in different file formats. The ETL should also be capable of validating and processing XBRL instance documents.
4.16 Data marts in specific subject areas, which are based on the existing BI (Business Intelligence) platform, may be used for data extraction and analyses on various datasets. Data may be presented through BI-based custom-built reports and ad hoc queries that business users themselves can prepare. Dashboards and visualisation tools may be developed for the use of top management and domain-specific senior management where relevant.
4.17 It is important to effectively use the micro and transactional data generated by the banking system in various applications such as forecasting inflation and growth, policy transmission and financial stability indicators close to real time. For this purpose, advanced analytics tools may be added to the existing data warehouse infrastructure and made available to internal users of the Bank. The existing BI platform may also be augmented suitably.
Activities between staff and vendors
4.18 The entire system may be designed, developed, operated and maintained through two sets of people, viz., the Reserve Bank’s own staff and outsourced vendor personnel. The activities to be handled by internal staff are the following:
-
Define data elements
-
Maintain data element, channel and dissemination directories
-
Develop taxonomies for data in co-ordination with domain experts and vendor personnel
-
Standardise and maintain various code lists
-
Define micro datasets
-
Perform business-related data quality analyses
-
Finalise and produce statistics for dissemination
-
Perform exploratory and predictive analytics
-
Co-ordinate with data sources and internal data users on business information exchanges
-
Provide access authorisation
-
Provide training and feedback
4.19 Activities to be handled by vendor personnel may include the following:
-
Develop, test and implement new data collection instruments
-
Establish data channels and monitoring data channels
-
Monitor network connections
-
Follow up with data sources in case of delays and non-submissions
-
Support statistical data processing (run batch, edit, move, publish datasets)
-
Develop data mart, dashboard, report and ETL
-
Maintain technical documentation
-
Make arrangements for backup and business continuity
-
Maintain the entire technical infrastructure in co-ordination with OEMs
-
Handle other technical and operational matters
Chapter 5
Data Quality Management
Introduction
5.1 Data quality management is the primary objective of the data compiler, who looks at two important dimensions of quality, namely, completeness and accuracy and timeliness in every cycle of data compilation. Other dimensions, like arithmetical relationships, are part of the system designed for data collection and, therefore, reflect the overall quality of the particular data compilation system. The Committee observed that in the current setup the aspects relating to system design as well as regular collection and processing are dispersed. There are no uniform standards or procedures, thereby partly contributing to variation in the data quality across various systems. Management of data quality is a resource-intensive process and requires considerable expertise and continuity of approach.
International experience
5.2 The European Central Bank (ECB) has a well-documented quality assurance procedure relating to its statistical functions (ECB, 2008) and covers the quality assurance procedures for (i) collection of data, (ii) compilation and statistical analysis and (iii) data accessibility and dissemination policy. The document lists completeness checks, internal consistency, consistency across frequency of the same dataset, external consistency, revision studies, plausibility checks and regular quality reporting. The plausibility checks use the principles of statistical quality control to identify reported figures that are significantly different from the usual reporting pattern. ECB statisticians use ARIMA models to assess such plausibility for a number of data series. In a survey of central banks the Denmark National Bank found that central banks have streamlined ‘administrative work’ and ‘outlier evaluations’ and achieved better ‘follow-up communication’ by automating their data validation processes (Drejer, 2012).
Data validation
5.3 Since data integrity is the most important objective of information management, ensuring that only valid and correct data gets stored in the data warehouse is of utmost importance. The Committee identified the following validation rules which are classified in generic terms and recommends that such rules may be specified for each dataset in consultation with domain experts.
-
Mandatory / optional character for a variable/ data field.
-
Data value should conform to a specified acceptable ‘list of values’.
-
A particular arithmetic relationship between two or more variables should be satisfied.
-
In a list of records, each record should be unique, as per a given set of identification parameters.
-
Certain relationships among values of specified variables are not acceptable or should be invariably satisfied.
-
The value/aggregate of a set of variables should be within reasonable closeness to another variable.
-
The value of a variable beyond a certain limit, either in absolute terms or in relation to another variable, is prima facie implausible.
The validation rules of the last two types require domain knowledge and the application of judgment. Therefore, the role of domain specialists in implementing these quality rules will have to be worked out for various datasets. The proposed validation process is illustrated in the following diagram drawn from IFC Working Paper No.11.
5.4 The Committee recommends that common systems may be used for data collection, storage and compilation in the Bank. A unified approach across various domains provides an opportunity to procure and deploy a professional-grade tool for data quality as part of the data warehouse infrastructure.
Chapter 6
Data Dissemination Policy
International experience
6.1 Several prominent central banks and international organisations have placed their well-documented information dissemination policies in the public domain. For instance, the dissemination policy of the US Federal Reserve Board (2002) discusses the detailed guidelines for ensuring and maximising the quality, objectivity, utility and integrity of information disseminated by the Board. The guidelines discuss in detail the information collected from regulated entities, collected through surveys and obtained from other agencies.
6.2 The dissemination policy document of the Statistics Directorate of the OECD (2002) specifies the objectives of dissemination as (i) wide dissemination of data, (ii) building credibility through high-quality statistics and (iii) contributing to the development of a culture of informed decision-making. To meet these objectives, the dissemination policy lays out actionable principles such as (i) cost-effective dissemination methods, (ii) free access to the user community, (iii) co-operation among statistical agencies on a reciprocal basis and (iv) a flexible pricing policy for academics and NGOs, in respect of the priced statistical publications.
6.3 The ECB’s quality assurance procedure (2008) for statistics also covers the data accessibility and dissemination policy. It states that the objective of the ECB statistical function is to provide the best possible service to internal users, national central banks (NCBs), market participants and the general public. Towards this end, the policy discusses adherence to the IMF’s SDDS, publication of data as per the pre-announced release calendar through press releases and statistical publications, online access to data via the ECB website, statistical data warehouse and a real-time database.
6.4 The Reserve Bank of India being the primary source for generating banking and financial statistics is required to disseminate the same to the public and share it with international institutions such as the BIS and the IMF. For efficient and effective sharing of information with international institutions, the Committee recommends that a separate web portal may be set up based on the SDMX (Statistical Data and Metadata Exchange) standard. An SDMX-based web portal will provide users with the flexibility to traverse through the list of variables and generate the required time series outputs. It will also help automate the sharing of information with other agencies by publishing the datasets through web services. Overall, such an SDMX-based portal will greatly enhance end-user experience in accessing data from the Bank.
6.5 The main objectives of dissemination are the following: (i) information should facilitate better decision-making for both the regulator and market participants, (ii) data availability should be improved to encourage and facilitate research and (iii) legal and contractual obligations for data confidentiality and privacy have to be meticulously followed. The data dissemination policy and practices of the Bank have evolved considerably in the past few years, with increasing use of the web channel and a gradual reduction in the number of printed copies of publications. The Committee recommends that the dissemination should be based on the principles of transparency, comprehensiveness, relevance and timeliness, almost in real time, with a view to improving public understanding through the official website. The Committee felt that the Bank does not have a comprehensive data dissemination policy in a well-documented format. The Committee, therefore, recommends that a dissemination policy for the Bank should be formulated and used as a guiding principle in disseminating or sharing information with various classes of users.
6.6 The dissemination policy needs to clearly distinguish between (i) data collected and compiled by the Reserve Bank from regulated/ other institutions, and (ii) data received by the Reserve Bank from other official/ authoritative sources for its functional use. Where the information is received by the Reserve Bank, there is a need to ensure consistency between the original source and the data with the Reserve Bank. There is also a need to include a disclaimer that cautions the user about the secondary nature of the data. If the Bank decides to disclose granular data that are collected under a confidentiality assurance for a certain purpose, the data should not include the identity or make it possible to indirectly identify the entity to which the data pertain. Wherever aggregate data are published, the granular data may be provided to users through an online query mechanism at the lowest possible level of aggregation, so that detailed analysis is possible without compromising the confidentiality condition.
6.7 Information collected under new surveys (where the initial efforts would be to improve coverage, put in quality checks and refine the methodology to make them consistent with the survey objective) may be released in the public domain, along with access to granular data on demand, after they stabilise and the Bank is satisfied with the representativeness of the results. Data should not be provided for periods where consolidated results are not published.
Principles of dissemination of the statistical requirements and metadata
-
The Reserve Bank may disseminate its statistical requirements from banks and other regulated entities through its public website at a particular periodicity.
-
The schedule for dissemination, along with frequency, may be disclosed in the form of a structured ‘Statistical Release Calendar’.
-
The formats, code lists, validation checks and other technical information required for information submission may also be placed in the public domain.
-
Definitions and other information required to clearly identify and interpret the data may also be provided along with the data.
-
The methodology used for collection, compilation, estimation and other characteristics of statistics may also be placed in the public domain and kept up-to-date.
Review of dissemination practices
6.8 At present, information is disseminated through three major channels, viz. (i) statistical publications, (ii) statistical/ press releases on the Reserve Bank website and (iii) the Database on Indian Economy (DBIE). Of these, the statistical publications have been progressively integrated with the DBIE and the process is nearing completion. All major statistical publications, viz., Handbook of Statistics on Indian Economy, Monthly RBI Bulletin (Current Statistics Section), Weekly Statistical Supplement and other quarterly/ annual publications are being brought out directly from the DBIE and the data are also available in a time series format.
6.9 As the coverage and periodicity of these publications have evolved over the years, there is some overlap in these publications. Sometimes, the overlap takes the shape of inconsistency due to different reference periods being followed by the publications for the same or similar variables, thereby creating avoidable confusion for users of the data. Therefore, the Committee recommends that a review of the coverage of the statistical publications may be undertaken internally by the Reserve Bank, with a view to streamlining the process and to remove/ reduce overlaps. The possibility of bringing out a single annual web-based publication, in addition to the Handbook, that covers the contents of all other annual statistical publications may be considered. Similarly, the quarterly releases that cover banking data and survey data can be combined into a single web-based statistical quarterly bulletin for web release, with identified contents and fixed release dates.
6.10 Another area that needs to be rationalised is the release of data at daily, weekly and fortnightly intervals through press releases. These releases are well-structured data releases and do not carry any other analysis. Therefore, it would be more convenient to separate them from other press releases and club them under a separate head, ‘Statistical Releases’, for easier access to historical data.
6.11 In addition to the original data series, the Reserve Bank may also consider providing select macroeconomic time series with value additions, such as series with a common base and seasonally adjusted series, along the lines of other central banks/ international organisations. As there is no unique method to arrive at the adjusted/ computed data series, these should be provided only as an additional facility, along with proper disclosure of the procedures followed in arriving at the computations/ derivations, so that users can make an informed decision to use the computed data or perform the computations themselves.
Data-sharing for research purposes
6.12 In general, external users from financial markets, the corporate world, academia or the general public may get access to the data available with the Reserve Bank within the framework of the dissemination policy outlined above. However, there may be instances where the Bank will find it necessary to conduct collaborative research with identified external researchers. In such instances, the Bank may decide to share data at a more granular level under specific confidentiality conditions.
Chapter 7
Governance and Management Structure
Organisation structure for information management
7.1 The present organisation of information management in a decentralised manner is not amenable to the implementation of uniform policies and standardisation of systems for information in the Bank. There is a clear need to put in place an appropriate governance structure for information management. This was also recognised by the High-level Committee on IT Vision (Chairman: Dr. K. C. Chakrabarty), which recommended that the Bank should identify and assign the role of Chief Information Officer (CIO) to a senior official. The CIO would be responsible for managing the information assets of the organisation, including data, and implementing data governance and the information governance framework.
7.2 The Committee is given to understand that the IT Sub-Committee of the Central Board also deliberated on the issue and has made recommendations on the role and responsibilities of the Chief Information Officer, which are broadly as follows:
• Policy
-
To frame and maintain various policies related to information management and governance: data management policy, access control policy and data dissemination policy
-
To formulate standards for data across different information domains in the Reserve Bank following international best practices
-
Set up a regular data quality assessment process and its implementation across various information domains
• Co-ordination
-
Co-ordinate with stakeholders in information management, such as information suppliers, users at various levels, IT managers and technology partners
-
Co-ordinate with the Chief Technology Officer (CTO) and the Chief Information Security Officer (CISO) to ensure the availability of proper technology and tools for information management/ security on an on-going basis, in line with emerging requirements
• Functional
- Manage the information resources of the entire organisation
7.3 The Committee concurs with the above views and recommends that the entire data requirement of the Reserve Bank should be managed centrally for coherence, integrity, consistency and accountability. The responsibilities of the information management unit should broadly be along the following lines:
-
Set up data governance policies
-
Maintain master data and data standards
-
Manage information resources (acquisition, storage, reports, dissemination) with IT tools and platforms
-
Provide feedback to user departments on data quality issues
-
Implement data quality based on the validation rules provided by the identified nodal business unit
-
Develop new modules as per user requirements
7.4 It is also recognised that given the wide scope of responsibilities envisaged under the new information management framework and several additional activities to ensure the integrity, consistency and accessibility of the information, the CIO will have to be assisted by sufficient manpower. The Committee suggests that the function of information management may be organised under an independent Information Management Unit (IMU) carved out of the current Department of Statistics and Information Management (DSIM). The roles and responsibilities of the proposed Information Management Unit are given in Annex 3.
7.5 The mandate of the CIO spans the information needs of the entire organisation. This will require consultations with users and stakeholders at various levels, including the highest levels in different functional areas. The process of formulating and executing information-related polices that affect the entire organisation will have to be participative and consultative. Therefore, the Committee recommends that a Policy Group may be formed with Heads/ Chief General Managers (CGMs) of select policy, functional and research departments as its members to advise the IMU.
7.6 The manpower in the Information Management Unit should predominantly have statistics and information technology (IT) as their core competency. The staff associated with information management in regulatory, supervisory and research departments may move to the IMU in a phased manner concurrently with the transfer of data-related activity from these departments to the IMU. In addition, there will be a need to post some officers in the unit who have knowledge of economics and experience in regulation and banking supervision to ensure that the information needs of the Bank for all its functions are well understood and met on a continued basis. The broad organisational structure is shown in Chart 2.
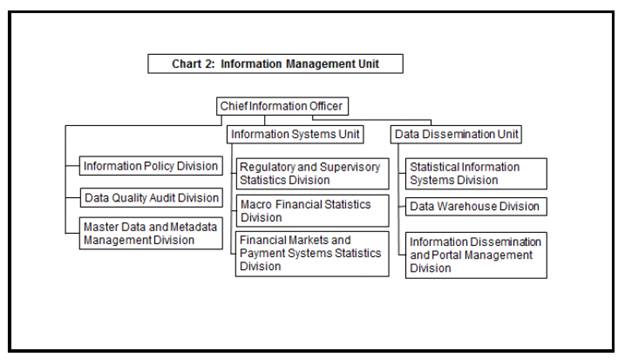
7.7 Each unit can be headed by a senior officer in Grade F, besides the Chief Information Officer. Each division can be headed by a Director in Grade D/E. Each division should have 5–6 officers with the necessary background and expertise. While several positions can be filled by redeploying the existing staff, additional positions may need to be created.
Chapter 8
Summary of recommendations
8.1 The recommendations of the committee are presented in this chapter.
Data Gaps
8.2 The Committee recommends that the data warehouse (DW) should be converted into an enterprise-wide data warehouse (EDW) where all the key data including supervisory data should reside without duplication. (Para No: 2.3)
Macro-financial statistics
8.3 Regarding macro-economic statistics, the Committee recommends compilation of the following indictors through co-ordination with the relevant government agencies.
-
Producer’s price index, services output, services price index and agricultural commodity futures prices. (Para No: 2.4)
-
A quarterly ‘labour force survey’ in India to provide internationally comparable employment/unemployment statistics. (Para No: 2.5)
-
A quarterly sample survey for collection of data on urban wages across broad industrial groups. (Para No: 2.6)
-
Construction and release of a survey of housing at a quarterly frequency. (Para No: 2.7)
-
A survey on the outlook for the services sector. (Para No: 2.8)
-
A survey on household indebtedness. (Para No: 2.8)
-
The scope and coverage of financial soundness indicators (FSI) should be expanded to other segments of the financial sector. (Para No: 2.9)
-
Quarterly flow-of-funds accounts starting with the financial sector. (Para No: 2.10)
-
A biannual survey to provide data on access, quality, usage and welfare impact of various financial services and products. (Para No: 2.14)
Regulatory and Supervisory Statistics
8.4 Committee recommends that the envelope of data elements across returns should be rationalised and the supervisory data should be moved to the enterprise-wide data warehouse (EDW) with appropriate accession rights for users. (Para No: 2.16)
8.5 The Committee recommends that the UCB and NBFC sector returns may be rationalised by identifying major data elements. (Para No: 2.20, 2.21)
8.6 The Committee recommends that possibility of including UCBs under the MOF may be explored. Further, MOF-like structure may also be designed to maintain branch information on NBFCs. (Para No: 2.13)
8.7 The Committee recommends an online data collection mechanism for larger NBFCs and Tier II UCBs. (Para No: 2.20, 2.22)
Data Model for Information System
8.8 The Committee was of the view that for primary data it would be optimal to dissolve the returns into data elements, which can be organised with relevant attributes and dimensions in the form of datasets of logically coherent data elements, for the collection of data. Regarding datasets that are secondary in nature, the input data structure is to be taken as available. (Para No: 3.2)
8.9 The Committee recommends three generic data collection structures: (i) aggregated data, (ii) distributed aggregates and (iii) granular data, depending on the relevance of data at different operational hierarchies. (Para No: 3.3)
8.10 The Committee also suggests that the values of various attributes and dimensions may be standardised to enable the collation of data from different domains. (Para No: 3.6)
8.11 The Committee recommends that it will be useful to prepare a Data Element Directory that lists of data elements to be collected from various constituencies with their related attributes. (Para No: 3.7)
8.12 The data element, data dissemination and data channel directories should be maintained centrally in a proper database format so that they can be accessed by data managers, data users and software developers. (Para No: 3.9)
Technology and Design
8.13 In addition to the existing software tools, the Reserve Bank may also acquire tools for: (i) a portal for Micro and SDMX-based Macro data dissemination and for managing the metadata directory, data flow channels and code lists, (ii) database for managing large micro datasets and (iii) data quality tools. (Para No: 4.6)
8.14 Bank-level aggregates, for which XBRL taxonomies and validation rules have been made available, should be submitted through XBRL instance documents generated directly from the central repositories of the banks without any manual interventions. (Para No: 4.8)
8.15 For granular micro (account/lowest unit-level data) and transactional multi-dimensional data, the Reserve Bank should develop and provide specific details of RDBMS/text file structures along with standardised code lists and basic validation rules. Using the secure network connections between the RBI server and the bank’s ADF server, the contents of the dataset may be pulled (ETL) or pushed (SFTP) and loaded onto the RBI’s server in an automated manner. (Para No: 4.9)
8.16 Effective Data Flow Monitoring System (DFMS) should be developed based on the Data Channel Directory to monitor data channels that connect various internal and external data sources and to ensure timely data flow in these channels through automatic or manual follow-up processes. (Para No: 4.12)
8.17 Big-data architecture may be considered for micro and transactional datasets considering their high volume, velocity and multi-dimensional nature. (Para No: 4.13)
8.18 The ETL should be capable of validating and processing XBRL instance documents. (Para No: 4.15)
8.19 Advanced analytics tools may be added to the existing data warehouse infrastructure and made available to internal users of the Bank. The existing BI platform may also be augmented suitably. (Para No: 4.17)
8.20 The entire system may be designed, developed, operated and maintained through two sets of people, viz., the Reserve Bank’s own staff and outsourced vendor personnel. (Para No: 4.18)
Data Quality Management
8.21 The Committee recommends that validation rules may be specified for each dataset in consultation with domain experts. (Para No: 5.3)
8.22 The Committee recommends that common systems may be used for data collection, storage and compilation in the Bank. A single tool across various domains provides an opportunity to procure and deploy a professional-grade tool for data quality, as part of the data warehouse infrastructure. (Para No: 5.4)
Data Dissemination Policy
8.23 The Committee recommends that dissemination should be based on the principles of transparency, comprehensiveness, relevance and timeliness almost in real time with a view to improving public understanding through the official website. (Para No: 6.5)
8.24 The Committee recommends that a dissemination policy for the Bank may be formulated and used as a guiding principle in disseminating or sharing information with various classes of users. (Para No: 6.5)
8.25 Wherever aggregate data are published, the granular data may be provided to users through an online query mechanism at the lowest possible level of aggregation, so that detailed analysis is possible, but without compromising the confidentiality condition. (Para No: 6.6)
8.26 The Committee recommends that the coverage of the statistical publications may be reviewed internally by the Reserve Bank to streamline the process and to remove/ reduce overlaps. (Para No: 6.9)
8.27 The Committee recommends that it will be more convenient to separate press releases that focus on specific data from other press releases, which should be clubbed under the separate head of ‘Statistical Releases’ for easier access to historical data. (Para No: 6.10)
Governance and Management Structure
8.28 The Bank should identify and assign the role of Chief Information Officer (CIO) to a senior official and recommends that the entire data requirements of the Reserve Bank should be managed centrally for coherence, consistency and accountability. (Para No: 7.3)
8.29 The Committee suggests that function of information management may be organised under an independent Information Management Unit (IMU) carved out of the current Department of Statistics and Information Management (DSIM). (Para No: 7.4)
8.30 The Committee recommends that a Policy Group may be formed with Heads/ CGMs of select policy, functional, and research departments as its members to advise the IMU. (Para No: 7.5)
8.31 The manpower in the Information Management Unit should predominantly have statistics and information technology (IT) as their core competency. There will be a need to post some officers in the unit with knowledge of economics and experience in regulation and banking supervision to ensure that the information needs of the Bank for all its functions are well understood and met on a continued basis. (Para No: 7.6)
8.32 Each unit can be headed by a senior officer in Grade F besides the Chief Information Officer. Each division can be headed by a Director in Grade D/E. Each division should have 5–6 officers with the necessary background and expertise. (Para No: 7.7)
References
Drejer, P. A. (2012): Survey on Practices in Optimizing Data Checking. Work Note - available upon request.
Drejer, P. A. (2013): Optimizing Checking of Statistical Reports, IFC Working Papers No 11, Irving Fisher Committee on Central Bank Statistics, Bank for International Settlement.
European Central Bank (2008): Quality Assurance Procedures within the ECB statistical function, ECB website.
http://www.ecb.europa.eu/pub/pdf/other/ecbstatisticsqualityassuranceprocedure200804en.pdf
Federal Reserve Board (2002): Guidelines for Ensuring and Maximizing the Quality, Objectivity, Utility, and Integrity of Information Disseminated by the Federal Reserve Board, http://www.federalreserve.gov/iq_guidelines.htm
IMF Staff and the FSB Secretariat (2009): The Financial Crisis and Information Gaps, Report to the G-20 Finance Ministers and Central Bank Governors.
OECD (2002): The OECD Dissemination Policy for Statistics, Statistics Directorate, OECD website. http://www.oecd.org/std/fin-stats/2754297.doc
Reserve Bank of India (2009): Report of the Working Group on Surveys.
Reserve Bank of India (2010a): Approach Paper on Automated Data Flow from Banks.
Reserve Bank of India (2010b): Report of the High Level Committee for Preparation of the Information Technology Vision Document 2011-2017.
SDMX (2011): SDMX-ML: Schema and Documentation, version 2.0; Available at
http://sdmx.org/docs/2_0/SDMX_2_0_SECTION_03A_SDMX_ML.pdf
Annex 1
Composition of Sub-Groups and Terms of Reference
(I) Data Issues for Regulation and Supervision including Banking
Shri P. R. Ravi Mohan, CGM-i-C, DBS Chairperson
Shri Kajal Ghosh, CGM, SBI
Shri Anil Jaggia, CIO, HDFC
Shri Anujit Mitra, Director, DSIM, Co-ordinator
Dr. Y. K. Gupta, Director, DSIM
Shri S. Majumdar, Director, FSU
Smt. Nivedita Banerjee, RO, DSIM
Issues:
-
Review the list of returns for banking data for policy, regulation and supervision and suggest rationalisation;
-
Identify important data elements with consistent definitions;
-
Suggest datasets of appropriate granularity and periodicity.
(II) Data Issues in Monetary, Macroeconomic Analysis and Financial Stability
Dr. Susan Thomas, IGIDR, Chairperson
Prof. Pami Dua, Director, DSE
Dr. B. K. Bhoi, Adviser, MPD
Shri Sanjay Hansda, Director, DEPR
Dr. Abhiman Das, Director, DSIM
Dr. (Smt.) Nishita Raje, Director, FMD
Shri Ravi Shankar, Director, DSIM, Co-ordinator
Shri Ujjwal Kanti Manna, Asst. Adviser, DSIM
Shri Sanjay Singh, Assistant Adviser, FSU
Issues:
-
Review data availability for Monetary, Macro Economic Analysis and Financial Stability in the light of felt needs in policy analysis as also consistent with global best practices particularly following from G-20 / IMF initiatives;
-
Identify data sources and methodology for new datasets.
(III) Data Requirements from Co-operative sector and NBFCs and for Financial Inclusion
Shri A. K. Bera, CGM, UBD, Chairperson
Dr. A. R. Joshi, Director, DSIM (Now Adviser, DSIM)
Dr. Pradip Bhuyan, Director, DSIM, Co-ordinator
Shri Manish Parashar, DGM, DNBS
Shri Ashish Jaiswal, AGM, RPCD
Smt. Sonali Adki, Asst. Adviser, DSIM
Smt. Shweta Kumari, RO, DSIM
Smt. Sangeetha Mathews, RO, DSIM
Issues:
-
Review data availability for the co-operative sector (UCBs), NBFCs pertaining to regulation and supervision and for financial inclusion;
-
Identify data gaps;
-
Identify important data elements;
-
Suggest ways for online collection of data on a granular basis depending on technology preparedness and systemic importance.
(IV) Data Modelling, Validation and Dissemination and Data Governance Structures
Prof. Bimal Roy, Director ISI, Chairperson
Shri Kajal Ghosh, CGM, SBI
Prof. Aditya Bagchi, ISI
Dr. Pinakpani Pal, ISI
Dr. A. R. Joshi, Director, DSIM, Co-ordinator (Now Adviser, DSIM)
Shri N. Senthil Kumar, Director, DSIM
Shri Shiv Shankar, Asst. Adviser, DSIM
Shri Amar Nath Yadav, Asst. Adviser, DSIM
Smt. Jolly Roy, Asst. Adviser, DSIM
Shri Dhirendra Gajbhiye, Asst. Adviser, DEPR
Issues:
-
Define Generic Data Models for data collection, storage and metadata;
-
Review and suggest changes to the data validation procedures and data quality management;
-
Design dissemination policy, standards and modalities for aggregate as well as micro data;
-
Review dissemination practices through various channels, viz., web, publications, DBIE;
-
Centralised/ federated organisation of statistical production activity;
-
Create organisation structure for information management, role and responsibilities of CIO.
(V) Technology and Design aspects of Information Management Systems
Dr. A.K. Srimany, Adviser, DSIM, Chairperson
Prof. N. L. Sarda, IITB
Dr. A. S. Ramasastri, CGM-in-Charge /Shri P. Parthasarathi, CGM, DIT (Alternate)
Shri Anil Jaggia, CIO, HDFC
Shri N. Senthil Kumar, Director, DSIM, Co-ordinator
Shri Purnendu Kumar, Asst. Adviser, DSIM
Shri Shiv Shankar, Asst. Adviser, DSIM
Shri Sourajyoti Sardar, Asst. Adviser, DSIM
Issues:
-
Design high-level architecture for information management;
-
Assess standards for granular data acquisition, push/pull technology, XBRL and other standards as compatible with banks’ source system;
-
Define architecture for statistical data processing;
-
Design architecture for micro and aggregated data management and extraction;
- Identify core activities of information management to be handled by internal staff and the out-sourcing strategy.
Annex 2
Element Directory Structure
Domain Master |
Id |
Description |
|
|
Subject area Master |
Id |
Description |
Domain |
|
|
|
Concepts Master |
ID |
Description |
Subject Area |
|
|
|
Data Element Master |
ID |
Name |
Definition |
Concept |
Type |
Nature |
Base Validation |
Arithmetic Relations |
Cross-Checks |
|
|
|
|
Amount Outstanding |
Leaf |
>0, <0, >=0, <=0 |
< = > f(some elements) |
Fill element-id |
|
|
|
|
Number of Units |
Fully Derived |
>0 |
eq 23+25 |
123=112 |
|
|
|
|
Inflow |
Partially Derived |
|
|
|
|
|
|
|
Outflow |
|
|
|
|
|
|
|
|
Growth Rate |
|
|
|
|
|
|
|
|
Ratio |
|
|
|
|
|
|
|
|
Index |
|
|
|
|
Data Channel Master |
Description |
Frequency |
Constituency |
Status |
Lag |
Channel |
Elements |
Fortnightly Return from SCBs |
Fortnightly |
Nationalised Banks |
|
|
SFTP push |
23,25,34,45… |
|
|
|
|
|
XBRL submission |
Annex 3
Broad Responsibilities of Information Management Unit
1. Information Policy Division
-
Data governance for the organisation’s overall needs
-
Overall policy for information management, acquisition, access, privacy, quality and dissemination
-
Co-ordination with data suppliers, users and other stakeholders
-
Introduction of new data collection elements, datasets and regular review of need/ redundancies
-
Management of vendor resources across various software, maintenance and production support activities
2. Data Quality Audit Division
-
Monitor the data quality status of various information channels
-
Data quality audit of information suppliers relating to statistical processes
-
Manage, collate and update data validation rules for various information domains
3. Master Data and Metadata Management Division
-
Define standard master data for all dimensions and classifications used in the information system of the Bank across all domains
-
Align the master data with international / national standards
-
Collect, maintain and manage metadata for all relevant domains of information
-
Track changes in definitions and methodologies and appropriately advise other units to incorporate them in a smooth and non-disruptive manner
-
Ensure that technical and user documents are prepared and maintained in an updated manner
Information Systems Unit
4. Regulatory and Supervisory Statistics Division
-
Data and reports requirements of banking regulatory and supervisory departments
-
Data and reports requirements of regulated entities other than banks
-
Data and reports requirements of foreign exchange regulations
5. Financial Markets and Payment Systems Statistics Division
-
Data and reports requirements of foreign exchange and other financial markets
-
Data and reports requirements of payment and settlement systems
-
Data and reports requirements of core banking system of the Reserve Bank and other transaction systems related to banking and currency issue functions
6. Macro Financial Statistics Division
-
Data and reports requirements from the macroeconomic statistical data such as output, prices, trade and employment.
-
Data and reports requirements from banking and financial sector related to money, banking, savings, and flow-of-funds.
Data Dissemination Unit
7. Statistical Information Systems Division
-
Management of information systems relating to data acquisition from regulated and other corporate bodies and online data procurement from other institutions
-
Development of taxonomies, datasets, and submission/ acquisition platforms, portals and other related software tools
-
Co-ordination with data suppliers, users and other stakeholders
-
Vendor management relating to development, maintenance and support of software applications and tools
8. Data Warehousing Division
-
Development of software systems relating to data storage, warehousing and archival
-
Technology tools required for all related systems
-
Management of the regular operations of related software and hardware resources
-
Co-ordination with the DIT for related technical infrastructure
9. Data Dissemination Portal Division
-
Design and specification of requirements of the data dissemination portal, executive dashboards and other electronic dissemination channels
-
Co-ordination with the Reserve Bank main website team for seamless integration of all data dissemination
-
Data dissemination through alternate electronic platforms/ devices through specific user interfaces
-
Manage dissemination of reports for internal users in the Bank, along with proper access control
-
Overall responsibility for electronic data dissemination to all users, including institutional users such as BIS, the IMF, the Government and banks
-
Bring out regular statistical publications of the Bank, namely, Handbook of statistics, Monthly Bulletin and Weekly Statistical Supplement
Abbreviations
ADF |
Automated Data Flow |
ARIMA |
Autoregressive Integrated Moving Average |
BC |
Business Correspondents |
BFS |
Board for Financial Supervision |
BI |
Business Intelligence |
BIS |
Bank for International Settlements |
BO |
Business Objects |
BSR |
Basic Statistical Returns |
CBS |
Core Banking Solution |
CDS |
Credit Default Swap |
CGM |
Chief General Manager |
CIO |
Chief Information Officer |
CISO |
Chief Information Security Officer |
CSO |
Central Statistics Office |
CTO |
Chief Technology Officer |
DBIE |
Database of Indian Economy |
DBOD |
Department of Banking Operations and Development |
DBS |
Department of Banking Supervision |
DEPR |
Department of Economic and Policy Research |
DFMS |
Data Flow Monitoring System |
DGI |
Data Gap Initiative |
DIT |
Department of Information Technology |
DNBS |
Department of Non-Banking Supervision |
DSB
DSIM |
Department of Supervision- Banking
Department of Statistics and Information Management |
DW |
Data Warehouse |
ECB |
European Central Bank |
EDW |
Enterprise Data Warehouse |
ETL |
Extraction, Transformation and Loading |
FI |
Financial Inclusion |
FID |
Financial Institutions Division |
FSI |
Financial Soundness Indicator |
HSUI |
Housing Start-Up Index |
IDRBT |
Institute for Development & Research in Banking Technology |
IFC |
Irving Fisher Committee (on Central Bank Statistics at BIS) |
IIT |
Indian Institute of Technology |
IMF |
International Monetary Fund |
IRISc |
Integrated Risk and Impact Scoring (Model) |
ISI |
Indian Statistical Institute |
IT |
Information Technology |
MIS |
Management Information System |
MOF |
Master Office File |
NBFCs |
Non-Banking Financial Companies |
NBFCs-D |
NBFCs - Deposit taking |
NBFCs-ND |
NBFCs - Non-deposit taking |
NBFCs-ND-SI |
NBFCs- Non-Deposit taking - Systemically Important |
NBS |
Non-Banking Supervision |
NCB |
National Central Bank |
OECD |
Organisation for Economic Co-operation and Development |
OEM |
Original Equipment Manufacturer |
OLAP |
On-line Analytical Processing |
ORFS |
Online Returns Filing System |
PCGM |
Principal Chief General Manager |
RBI |
Reserve Bank of India |
RBS |
Risk-Based Supervision |
RDBMS |
Relational Database Management System |
RPCD |
Rural Planning and Credit Department |
RRB |
Regional Rural Bank |
RTGS |
Real Time Gross Settlement |
SCB |
Scheduled Commercial Bank |
SDDS |
Special Data Dissemination Standard |
SDMX |
Statistical Data and Metadata Exchange |
SFTP |
Secure File Transfer Protocol |
SSM |
Senior Supervisory Manager |
StCB |
State Co-operative Bank |
UBD |
Urban Banks Department |
UCB |
Urban Co-operative Bank |
XBRL |
eXtensible Business Reporting Language |
XSD |
XML Schema Definition |
|