Saurabh Ghosh and Snehal Herwadkar*
We analyse the effect of portfolio flows on various segments of the Indian financial
markets over the decade preceding the global financial crisis. The correlation analysis and the
causality test results suggest that portfolio flows cause changes in equity prices and exchange
rates. In the short run, the VAR and impulses response functions indicate that a positive shock
to net FII flow generally result in increase in equity prices, exchange rate (INRUSD)
appreciation and a decline in interest rates. The magnitude of these responses dampens over
time and converges towards equilibrium path. In the long run, the parameters of Autoregressive
Distributed Lag (ARDL) Model indicate the existence of an equilibrating relation. The
magnitude and direction of long term coefficients generally support the short run findings.
The negative and significant error correction term, on the other hand, indicates movements
towards long run equilibrium and the resilience of Indian financial markets.
JEL Classification : G11, G14
Keywords : Portfolio flow, Financial Markets
I. Introduction
The decade immediately preceding the global financial crisis
(1998-2008) had witnessed an exponential increase in international
capital movements, especially in the emerging markets. India was
no exception to this phenomenon, where with the ushering of
economic liberalisation and globalisation there was a large increase
in the portfolio inflows. The favourable demographic characteristics
(Mohan, 2004), growth potential, scope of diversification and
economic policies also influenced India’s share in capital flows
among Emerging Market Economies (EMEs). Consequently, the Indian financial markets started getting increasingly integrated with
the global financial market (Sinha and Pradhan, 2008). Anecdotal
evidence suggest that global factors started influencing domestic
markets through the portfolio channel over the last decade.
It is well established in the literature that financial markets suffer
from contagion – both foreign and domestic [Lagoarde and Lucey
(2007)]. While the former refers to a shock to a country’s financial
markets caused by changes in markets of another country, the latter
refers to turbulence in one market spilling over to other market
segments of the same country. These movements across financial
markets are often synchronous in nature, particularly in EMEs. These
two effects often reinforce each other and amplify the problem.
The East Asian crisis clearly indicated the vulnerability of an open
economy to sudden changes in portfolio flows. With gradual progress
towards external sector liberalisation and increasing capital inflows,
the issues relating to the resilience of Indian financial system in the
face of strong and volatile capital flows attained increasing importance.
The present study is an attempt to address these issues. It aims to
analyse the effects of portfolio flows across different financial markets.
Specifically, this study has two objectives: it attempts to model the short
run and long run relationship between the portfolio flows and different
segments of the financial market. Second, it intends to assess the impact
of sudden changes in such flows on various segments of the financial
markets. This paper is organised as follows: Section II presents a brief
survey of literature, Section III describes the data, Section IV analyses
the empirical findings and Section V draws conclusions.
Section II
Literature Survey
One of the major arguments in favour of the financial
liberalisation and globalisation is that it facilitates greater economic
growth. Many economists also believe that free capital flows will
lead to a more efficient allocation of resources (e.g. Kim and Singal,
2000). Considering the beneficial role played by capital flows, early research interest was focused on the determinants of such flows.
For instance, studies by Taylor and Sarno (1997), Brennan and Cao
(1997), Mody and Murshid (2005) and Lagoarde and Lucey (2007)
concentrated on the determinants of the portfolio investment and
their impact on the receiving economies. Their empirical findings,
though not unanimous, generally point towards productivity growth
and allocative efficiency of the capital flows.
Towards the end-1990s, especially after the outbreak of
economic crisis in South East Asian countries, issues such as
volatility of the ‘hot’ capital flows and their impact on the recipient
country, problems relating to contagion etc. have increasingly
occupied the centre-stage of academic research. Subsequently, many
policy makers and economists became skeptical not only about the
benefits of free flows, but also viewed uncontrolled capital flows as
risky and destabilising. Krugman (1998) underlined the problem of
moral hazard in financial intermediaries and noted that it can lead
to over-investment at the aggregate level, overpricing of assets and
vulnerability of such economies to financial crises. Subsequent
studies (e.g. Dabos and Juan-Ramon (2000)) concentrated on the
intricate relationship between capital flows and financial markets.
The recent literature has acknowledged the risks associated with the
capital flows and indicated that the most effective way to deal with
capital inflows would be to deepen the financial markets, strengthen
financial system supervision and regulation, improve capacity and
implement sound macroeconomic / financial sector policies. These
actions will help in increasing the absorptive capacity and resilience of
the economies and financial systems to the risks associated with large
and volatile inflows. To quote Mohan (2009), ‘It is a combination of
sound macroeconomic polices, prudent debt management, exchange
rate flexibility, the effective management of the capital account, the
accumulation of appropriate levels of reserves as self-insurance and
the development of resilient domestic financial markets that provides
the optimal response to the large and volatile capital flows to the
EMEs. How these elements are best combined will depend on the
country and on the period: there is no ‘one size fits all’’.
Since the beginning of 2000s, empirical studies have concentrated
on capital flows in India and its impact on domestic macro variables.
In this context a study by Dua and Sen (2005) found that the real
effective exchange rate is cointegrated with the level of capital flows,
volatility of the flows, high-powered money, current account surplus
and government expenditure. The results reported by Trivedi and Nair
(2000) indicate that the returns and volatility in the Indian markets
emerge as the principal determinants of FII investments. D’souza
(2008) noted that the difference between the capital flows to India
as compared with other EMEs are that (a) they are associated with
a deteriorating current account position rather than improving one
and (b) the extent of financial outflows have only partially offset the
capital inflows. The author also notes that capital flows in India have
been associated with a buoyant stock market and a rise in investment
and interest rates in the economy.
While most of the previous empirical studies considered impact of
capital flows on the macroeconomic variables or on a particular market
(e.g. stock market / exchange rate market etc.) our study attempts to
integrate the major financial markets and evaluate the market reaction
to a shock in capital flows. In other words, the emphasis is on market
dynamics and resilience to uncertain and volatile flows rather than
analysing the determinants or the effect of capital flows on domestic
variables. It assumes significance as India moves towards greater
capital account liberalisation (A brief write-up on India’s approach
to capital flows is reported in the Annex I), and faces the challenges
of coping with large, volatile and uncertain capital flows.
Section III
Data
The major data-source for this study is Handbook of Statistics
on the Indian Economy (HBS). Our study uses the monthly time
series data on financial variables over the decade just before the
outbreak of global financial crisis(April 1998 to March 2008). The
period 2008-09, which was mainly characterised by exterme events and freezing of financial markets, was not included in the empirical
analysis as it may distort the general trends observed in the financial
markets. However, results obtained were compared with reference to
this period, which serves as a robustness test.
We used rupee dollar (INRUSD) exchange rate to represent
the movements in forex market and yields of benchmark 10-year
Government of India securities (GSEC) as a representation of gilt
movements. While for the equity market, monthly average data
for BSE Sensex (Sensex) has been used, the monthly average call
/ notice rate (CALL) is used for representing the interbank money
market. The monthly data on net investments in debt and equity
by foreign institutional investors (NETFII) were gathered from the
Securities and Exchange Board of India web-site. Table-1 reports the
descriptive statistics for these variables.
It may be noted that the FII flows in Indian markets consist of
investments in equities as well as in debt instruments. Even though
the FII investments in equities have consistently remained much
higher than the same in debt instruments, the FII investments in debt
have almost doubled during 2007-08 as compared with 2006-07.
Unlike some of the earlier studies, in view of the growing investment
of FIIs in debt instruments, we have used both of these flows in the
present paper.
Table 1: Descriptive Statistics |
|
Net FII |
Sensex |
INRUSD |
Call |
GSEC |
1 |
2 |
3 |
4 |
5 |
6 |
Mean |
1983.94 |
5907.94 |
45.10 |
6.78 |
8.52 |
Median |
1088.87 |
4647.34 |
45.25 |
6.66 |
7.68 |
Maximum |
19515.29 |
15253.42 |
49.00 |
14.07 |
12.33 |
Minimum |
-8930.32 |
2866.55 |
39.66 |
4.29 |
5.11 |
Std. Dev. |
3280.08 |
3427.70 |
2.18 |
1.96 |
2.33 |
Skewness |
1.46 |
1.36 |
-0.13 |
0.99 |
0.33 |
Kurtosis |
9.87 |
3.62 |
2.40 |
4.22 |
1.72 |
Section IV
Methodology and Empirical Findings
IV.1 Basic Results
The objective of this paper is to study the interrelationship of
portfolio flows in India with other financial variables. Following the
standard time series methodology, we first check for stationarity of
these variables. This involves determining the order of integration of
each of the variables under consideration by employing one of the
unit root tests. In this paper we employ the widely accepted Phillips-
Perron (1988) t-test. The results are reported in Table 2 below.
The Phillips-Perron adjusted t-stat and their p-values indicate
that CALL and NETFII are both stationary at levels. The SENSEX,
GSEC and INRUSD rate are first difference stationary. The correlation
coefficients of the above variables are reported in Table 3. For
computing the correlation coefficients the non-stationary series are
included in the differenced form (to avoid spurious results), while the
stationary variables are in the levels.
Table 3 indicates that NETFII has a high positive coefficient
with changes in SENSEX and negative correlation coefficient with
other financial rate variables (CALL, GSEC and INRUSD). So, an
increase in NETFII could lead to an increase in SENSEX, reduction
in interest rates and put an appreciating pressure on INR.
IV.2 Causality Analysis
In the previous section we found some evidence of
contemporaneous relation between the financial variables with net capital inflow in India. This section examines the possibility of leadlag
relationship among these financial variables. For evaluating the
same we considered Granger causality test1. Table 4 reports the null
hypothesis, Granger F-Statistics and the probability values associated
with the F-statistics.
Table 2: Phillips-Perron Adjusted t-Statistics |
Null Hypothesis |
Phillips-Perron test statistic |
Level |
differenced |
Adj.t-Stat |
Prob. |
Adj. t-Stat |
Prob. |
1 |
2 |
3 |
4 |
5 |
CALL has a unit root |
-4.02 |
0.00 |
|
|
INRUSD has a unit root |
-2.13 |
0.23 |
-7.50 |
0.00 |
NETFII has a unit root |
-10.71 |
0.00 |
|
|
SENSEX has a unit root |
-0.23 |
0.93 |
-7.36 |
0.00 |
GSEC has a unit root |
-1.54 |
0.51 |
-9.30 |
0.00 |
Table 3: Correlation Coefficient |
|
NETFII |
CALL |
DSENSEX |
DGSEC |
DINRUSD |
1 |
2 |
3 |
4 |
5 |
6 |
NETFII |
1.00 |
-0.27 |
0.56 |
-0.11 |
-0.48 |
CALL |
-0.27 |
1.00 |
-0.21 |
-0.02 |
0.12 |
DSENSEX |
0.56 |
-0.21 |
1.00 |
-0.23 |
-0.38 |
DGSEC |
-0.11 |
-0.02 |
-0.23 |
1.00 |
0.09 |
DINRUSD |
-0.48 |
0.12 |
-0.38 |
0.09 |
1.00 |
Table 4 : Pair-wise Granger Causality Test |
Null Hypothesis: |
F-Statistic |
Probability |
1 |
2 |
3 |
DSENSEX does not Granger Cause NETFII |
0.01 |
0.99 |
NETFII does not Granger Cause DSENSEX |
2.57 |
0.08 |
DGSEC does not Granger Cause NETFII |
0.20 |
0.82 |
NETFII does not Granger Cause DGSEC |
0.91 |
0.40 |
CALL does not Granger Cause NETFII |
1.60 |
0.21 |
NETFII does not Granger Cause CALL |
0.57 |
0.57 |
DINRUSD does not Granger Cause NETFII |
1.38 |
0.24 |
NETFII does not Granger Cause DINRUSD |
2.66 |
0.11 |
DGSEC does not Granger Cause DSENSEX |
0.80 |
0.45 |
DSENSEX does not Granger Cause DGSEC |
1.07 |
0.35 |
CALL does not Granger Cause DSENSEX |
3.90 |
0.02 |
DSENSEX does not Granger Cause CALL |
0.32 |
0.72 |
DINRUSD does not Granger Cause DSENSEX |
0.93 |
0.40 |
DSENSEX does not Granger Cause DINRUSD |
0.10 |
0.91 |
CALL does not Granger Cause DGSEC |
2.25 |
0.11 |
DGSEC does not Granger Cause CALL |
0.13 |
0.88 |
DINRUSD does not Granger Cause DGSEC |
0.42 |
0.66 |
DGSEC does not Granger Cause DINRUSD |
0.15 |
0.86 |
DINRUSD does not Granger Cause CALL |
4.61 |
0.01 |
CALL does not Granger Cause DINRUSD |
6.64 |
0.00 |
Table 4 indicates that NETFII causes changes in SENSEX and
INRUSD. There are also empirical evidences of CALL causing changes
in SENSEX and Yield. The F-Statistics and the associated P-values
indicate bi-directional causality between CALL and INRUSD.
IV.3 Short Run Adjustments: Impulse Responses
In order to examine the directional impact and the time path of
a change in NETFII flows on other financial variables, we used a
basic five variable VAR model comprising of net FII flows (NETFII),
exchange rate (INRUSD), Stock indices (SENSEX), Call rate
(CALL) and benchmark yield (GSEC). The impulse responses trace
out the responsiveness of these variables to a shock to NETFII. The
level stationary variables were used in levels for the VAR, whereas
the difference stationary variables were used in difference form. The
order of the VAR was selected using the SBC criteria. A unit shock
was applied to the errors of NETFII equation, and the effects upon
the VAR system over time were noted. For generating the impulse
responses, we used orthogonalised (Choleski) impulse responses
to a unit standard deviation shock; Chart 5 reports these impulse
responses along with analytical standard error bands (represented by
the dotted lines).
A look at the impulse responses, over an eight-month period,
reveals two stylised facts. First, a shock in NETFII has a negative
impact on most financial variables. For instance, differenced
exchange rate initially declined, indicating appreciation of INR, due
to the increase in NETFII flows. The call rate also declined initially,
indicating surplus liquidity in the money market. The NETFII flows,
on the other hand, had a positive impact on the stock market, indicating
the important link between capital flows and the stock prices in India.
These results are consistent with the available literature / anecdotal
evidence on capital flows and markets in EMEs. The impact of such
impulses either dampened over time or hovered around the initial
values indicating the resilience of the financial markets in India in
the short run.
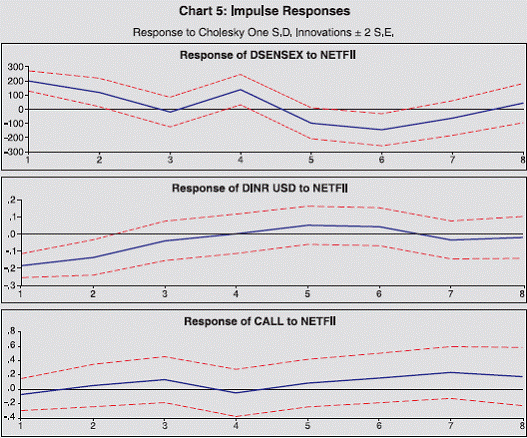 |
A simple VAR system and impulse responses are often
criticized because ordering of the equations in the VAR system plays a
role in the magnitude and direction of impulse responses. To ascertain
the robustness of the results, we cross-checked the impulse responses
through the Generalised impulses, as introduced by Pesaran and Shin
(1998), which in contrast to Choleski decomposition does not depend
on the VAR ordering. The impulse responses that are reported in
Annex Chart A2 confirm the above findings.
IV.4 Long Term Relation (Cointegration)
In this section we test for the existence of a long-run relationship
between NETFII and financial variables within a multivariate
framework. The general process for testing the same for a set of nonstationary
variables (of same order or integration) is by testing the
existence of cointegrating vector(s) using Johansen (1988) method.
However, the PP test (Table - 4) indicates that all variables under
consideration here do not have the same statistical properties (rather a combination of I(0) and I(1) variables). Therefore, in order to test for
the existence of any long-run relation among the variables, we used
Autoregressive Distributive Lag (ARDL) method and the bounds
testing approach to cointegration. The main advantage of ARDL
testing lies in the fact that it can be applied irrespective of whether
the variables are I(0) or I(1) [Pesaran et al.(1996)]. The test gives
asymptotically efficient long run estimates irrespective of whether
the underlying regressors are I(0) or I(1) process. The specification
of the ARDL model is as follows :
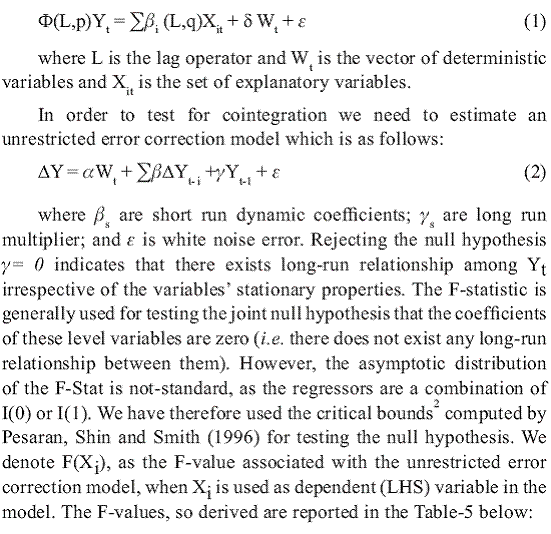 |
Table 5 : ARDL F-Statistics Indicating Long Term Relationship |
F(INRUSD) |
F(SENSEX) |
F(NETFII) |
F(GSEC) |
F(CALL) |
1.94 |
2.24 |
1.18 |
2.20 |
3.59 |
Given the number of variables (k=4) the relevant critical value
bounds for the present application at the 90 per cent level are given
by 2.2425 to 3.574. Since F(CALL) = 3.59 exceeds the upper bound
of the critical value band, we can reject the Null of no long-run
relationship among the variables under consideration.
The estimation of the long-run coefficients and the associated
error-correction model can now be accomplished using the ARDL
methodology. The order of the ARDL model was selected using both
the Schwarz Bayesian (SBC) and the Akaike Information (AIC)
criteria and the selected model (using SBC as well as AIC criteria)
was of ARDL(1,0,0,0,0) specifications. While the ARDL coefficients
are reported in the Annex Table A3 the estimates of the long-run
coefficients based on this model are summarised in Table 6 (column
2 and 3) below.
Column 4 and 5 of the Table 6 report the long term coefficients
derived from the augmented ARDL model. The augmented model
incorporates five additional dummy variables. The LAF Cap dummy
captures months (2007M3 – 2007M7) when the reverse repo (absorption) window of Liquidity Adjustment facility3 was capped
at Rs.3,000 crore and the money market rate plunged considerably
due to excess liquidity in the interbank market. The four end-quarterdummies
were incorporated to capture the seasonal patterns in the
Indian money market. The rate and volatility in the money market
generally increase during the end-quarter mainly due to advance
quarterly tax outflow from the system. The quarterly dummies
were incorporated in the augmented model to capture the seasonal
pattern in the money market. The dummy coefficient for March was
significantly different from zero at five percent level. It may be noted
from the above table that the point estimates in both the cases are
similar in magnitude and signs. They indicate that increasing GSEC
yield (rising cost of capital) and Sensex (booming capital market)
put upward pressure on money market rates. The capital flows, on
the other hand, have an easing impact on the money market rates.
This could be due to the fact that during the period of large capital
inflows, the central bank’s forex operations4 (purchase of dollar), at
times, increase liquidity in the domestic money market and therefore
have an easing impact on rates. The sign of INRUSD was found
to be positive. This could be because of the fact that the periods
characterised by INR depreciation are generally marked by large
capital outflows, forex operations (sell of dollars) and therefore
relatively tight liquidity conditions (higher money market rates). All
these coefficients were statistically significant at 10 per cent levels.
Table 6 : Long Run ARDL coefficients |
Regressor |
Coefficient |
T-Ratio[Prob] |
Coefficient |
T-Ratio[Prob] |
1 |
2 |
3 |
4 |
5 |
INRUSD |
0.2907 |
1.9082[.060] |
0.2898 |
1.9058[.060] |
SENSEX |
0.0002 |
2.9505[.004] |
0.0002 |
3.0451[.003] |
GSEC |
0.8060 |
8.4125[.000] |
0.8107 |
8.7525[.000] |
NETFII |
-0.0001 |
-1.8885[.062] |
-0.0001 |
-1.9032[.060] |
C |
-14.0444 |
-2.4970[.014] |
-14.1583 |
-2.6093[.010] |
T (LAF Cap) |
|
|
-0.5756 |
-.62697[.532] |
S3 (Mar) |
|
|
1.4144 |
2.3649[.020] |
S6 (June) |
|
|
-0.6085 |
-.98366[.328] |
S9 (Sep) |
|
|
0.0276 |
.046944[.963] |
R-Squ |
0.67 |
|
0.69 |
|
D-W Stat |
1.99 |
|
2.04 |
|
The error correction coefficient, (Table 7) estimated using the
same ARDL model was at 0.661(0.00), statistically highly significant
and had the correct (negative) sign, which suggested reasonable
speed of convergence to equilibrium5. The ECM coefficient for the
augmented model was also consistent with the former and indicated
the robustness of these models.
The above error correction model can also be used in forecasting
the changes in money market rates due to changes in capital flows
and changes in other financial market variables. To test the robustness of the model, forecast for 2008M4 to 2008M7 was done using both
the models (simple as well as Augmented ARDL models) which are
referred in Table 8 below:
Table 7 : ARDL Error Correction Model |
Regressor |
Coefficient |
T-ratio[prob] |
Coefficient |
T-ratio[prob] |
1 |
2 |
3 |
4 |
5 |
DINRUSD |
0.1921 |
2.5676[.012] |
0.1961 |
2.6318[.010] |
DSENSEX |
0.0001 |
2.8494[.005] |
0.0001 |
2.9907[.003] |
DGSEC |
0.5327 |
5.7160[.000] |
0.5487 |
5.8797[.000] |
DNETFII |
0.0001 |
-1.9624[.052] |
0.0001 |
-1.9575[.053] |
dC |
-9.2809 |
-2.3923[.018] |
-9.5820 |
-2.4866[.014] |
dT |
|
|
-0.3895 |
-.64023[.523] |
dS3 |
|
|
0.9572 |
2.4026[.018] |
dS6 |
|
|
-0.4118 |
-.98356[.328] |
dS9 |
|
|
0.0187 |
.046938[.963] |
ecm(-1) |
-0.66082 |
-7.8173[.000] |
-0.67677 |
-7.8603[.000] |
R-Square |
0.37 |
|
0.42 |
|
D-W Stat |
1.99 |
|
2.04 |
|
The root mean squares of forecast errors of the estimated period
compared favourably with that in the sample period. The RMSE of
the Augmented ARDL model was lower, but in line with the former,
which supports finding of the earlier models. The actual and estimated
values of call rates are plotted in the Annex Chart A-3.
Table 8 : Forecast Errors |
|
Model I |
Model II |
1998M4-2008M3 |
2008M4-2008M7 |
1998M4-2008M3 |
2008M4-2008M7 |
1 |
2 |
3 |
4 |
5 |
Mean |
0.000 |
-0.442 |
0.000 |
-0.254 |
Mean Absolute |
0.678 |
0.784 |
0.677 |
0.694 |
Mean Sum Squares |
1.350 |
0.661 |
1.252 |
0.636 |
Root Mean Sum Squares |
1.162 |
0.813 |
1.119 |
0.797 |
Section V
Conclusion
The literature so far is not unanimous about the movements in
the financial markets as a result of capital flows. The present paper
evaluates this ongoing debate in the Indian context. The results
indicate that net FII flows cause changes in equity (SENSEX) and
exchange rate (INRUSD). In the short run, a shock to net FII flow has
a positive impact on equity market and negative impact on money
market (CALL) rate, benchmark yield and exchange rate (indicating
Rupee appreciation). The impulse responses dampen over time.
The capital inflows and returns on equities generally have a
positive correlation for emerging markets. The empirical findings
of this study confirms the same for India over the last decade. One
of the major reasons for surplus liquidity in Indian money market
during 2004:04 to 2008:03 was large capital inflows and consequent
upward pressure on the Indian Rupee. The Central Bank’s forex
operations in the face of large capital inflows had a positive impact
on the domestic money supply and interbank liquidity. This in turn
put a downward pressure on the rates in the money market. In an
attempt to reduce surplus liquidity from the money market, the central
bank sterilised excess liquidity (through Open Market Operations
or Market Stabilisation Scheme), which influenced the rates in the
G-sec market. These calibrated policies have been captured in the
short run analysis of the present paper.
Over the long run, the result of the ARDL model generally supports
the short run dynamics. The bound test (of ARDL model) indicates
that there exists a long run relation between capital flows and financial
variables. The error correction term has a negative coefficient and
was found to be statistically highly significant, indicating reasonable
speed of convergence to the equilibrium. The long run coefficients
of the ARDL model for yield, exchange rate (increase indicate rupee
depreciation) and equity had a positive effect on call rate, while
the net FII inflow has a negative effect on the same. The long term
coefficients of the ARDL model and the negative and significant error
correction term, indicates the resiliance of Indian financial markets.
Finally, this study mainly concentrated on the pre-global
crisis period to evaluate the long term interaction between financial
variables during normal times. Though the impact of the recent
global crisis was rather muted for the Indian economy, some capital
outflows were witnessed during September-October 2008, with
concomitant pressure on the financial markets. However, because of
the pre-emptive policy measures and the resilience of the financial
system, the markets were back to normal by December 2008. While
the quick adjustment and resilience demonstrated by the markets
reinforce the empirical findings of our study, it also opens up several
areas for further research (e.g. contingency measures and the reaction
of various financial markets in response to such measures). With the
availability of longer time series data, these issues can trigger off
more focussed research on financial markets and capital flows, which
would serve as a useful guide both in refining operating procedures
and furthering financial markets reforms in India.
Annex I : The Indian Approach to Capital Flows : An Overview
Until the 1980s, India’s development strategy was focussed on selfreliance
and import-substitution. There was a general disinclination towards
foreign investment or private commercial flows. Since the initiation of the
reform process in the early 1990s, however, India’s policy stance has changed
substantially. India has encouraged all major forms of capital flows, though
with caution, in view of concerns for macroeconomic stability. The broad
approach to reform in the external sector after the Gulf crisis was delineated in
the Report of the High Level Committee on Balance of Payments (Chairman:
C. Rangarajan). The Report, inter alia, recommended a compositional shift
in capital flows away from debt to non-debt creating flows; strict regulation
of external commercial borrowings, especially short-term debt; discouraging
volatile elements of flows from non-resident Indians; gradual liberalisation
of outflows; and disintermediation of Government in the flow of external
assistance. In the 1990s, foreign investment has accounted for a major part
of capital inflows to the country. The broad approach towards foreign direct
investment has been through a dual route, i.e., automatic and discretionary,
with the ambit of the automatic route progressively enlarged to many sectors,
coupled with higher sectoral caps stipulated for such investments. Portfolio
investments are restricted to select players, viz., Foreign Institutional Investors
(FIIs). In respect of NRI deposits, some control over inflows is exercised
through specification of interest rate ceilings. In the past, variable reserve
requirements were stipulated to modulate such flows. At present, however,
reserve requirements are uniform across all types of deposit liabilities (see,
for instance, RBI, 2004b).
In connection with external assistance, both bilateral and multilateral
flows are administered by the Government of India and the significance of
official flows has declined over the years. Thus, in managing the external
account, adequate care is taken to ensure a sustainable level of current account
deficit, limited reliance on external debt, especially short-term external debt.
Non-debt creating capital inflows in the form of FDI and portfolio investment
through FIIs, on the other hand, are encouraged. A key aspect of the external
sector management has, therefore, been careful control over external debt
since 1990s (Reddy, 1998). India has adopted a cautious policy stance with
regard to short-term flows, especially in respect of the debt-creating flows.
In respect of capital outflows, the approach has been to facilitate direct
overseas investment through joint ventures and wholly owned subsidiaries.
Exporters and exchange earners have also been given permission to maintain
foreign currency accounts and use them for permitted purposes to facilitate
their overseas business promotion and growth. Thus, over time, both inflows
and outflows under capital account have been gradually liberalised.
Annex II
Annex II (Concld.) |
Table A3 :ARDL Estimate Results |
Regressor |
Coefficient |
T-Ratio[Prob] |
Coefficient |
T-Ratio[Prob] |
CALL(-1) |
0.3392 |
4.0123[.000] |
0.3232 |
3.7541[.000] |
INRUSD |
0.1921 |
2.5676[.012] |
0.1961 |
2.6318[.010] |
SENSEX |
0.0001 |
2.8494[.005] |
0.0001 |
2.9907[.003] |
GSEC |
0.5327 |
5.7160[.000] |
0.5487 |
5.8797[.000] |
NETFII |
0.0001 |
-1.9624[.052] |
0.0001 |
-1.9575[.053] |
C |
-9.2809 |
-2.3923[.018] |
-9.582 |
-2.4866[.014] |
T |
|
|
-0.3895 |
-.64023[.523] |
S3 |
|
|
0.9572 |
2.4026[.018] |
S6 |
|
|
-0.4118 |
-.98356[.328] |
S9 |
|
|
0.0187 |
.046938[.963] |
Notes :
* Saurabh Ghosh is an Assistant Adviser working in the Financial Markets
Department and Snehal Herwadkar is an Assistant Adviser working with the
Department of Economic Analysis and Policy, Reserve Bank of India. The views
expressed here are that of the authors alone and do not necessarily represent the
official policy stance of the Reserve Bank of India.
1 If the variable y1 causes y2, lag of y1 would be jointly significant in the
equation of y2. The joint significance of the lags were evaluated with the
help of Granger F-test and the lag lengths have been selected using the
minimum value of Schwarz – Bayesian Information Criteria (SBC).
2 Two sets of critical value are given. One set assuming that all variables
in the ARDL model are I(1), and other computed assuming all the variables
are I(0).
3 A tool used in monetary policy that allows banks to borrow money through
repurchase agreements. Repo (Reverse Repo) indicates injection (absorption)
of liquidity by central bank in (from) the banking system.
4 The exchange rate policy in recent years in India has been guided by the
broad principles of careful monitoring and management of exchange rates
with flexibility, without a fixed target or a pre-announced target or a band,
coupled with the ability to intervene, if and when necessary. The overall
approach to the management of India’s foreign exchange reserves takes into
account the changing composition of the balance of payments and endeavours
to reflect the ‘liquidity risks’ associated with different types of flows and
other requirements (First Quarter Review of Annual Monetary Policy for
the Year 2008-09).
5 The larger the error correction coefficient the faster is the economy’s
return to its equilibrium, once shocked.
References
Agarwal R. (1997), ‘Foreign Portfolio Investment In Some Developing
Countries: A Study of Determinants and Macroeconomic Impact’, Indian
Economic Review, vol. 32, issue 2, pages 217-229.
Alfaro, L., Chanda, A., Kalemli-Ozcan, S., Sayek, S. (2003), ‘FDI Spillovers,
Financial Markets and Economic Development’, IMF Working Paper No.
03/186
Brennan, M., Cao, H. (1997), ‘International Portfolio Investment Flows’,
Journal of Finance, December, 52(5): 1851-80.
Chakrabarti, R. (2001), ‘FII Flows to India: Nature and Causes’, Money and
Finance, Vol. 2, No. 7, October-December 2001
Dabos, M. and V. Juan-Ramon, 2000. Real Exchange Rate Response to Capital
Flows in Mexico: An Empirical Analysis, IMF Working Paper, WP/00/108,
June.
D’Souza, E. (2008), ‘Globalisation’s Underbelly: Capital Flows and Indian
Economy’, Economic and Political Weekly, August 30, 2008.
Detragiache, E., P. Gupta, (2004), ‘Foreign Banks in Emerging Market Crises:
Evidence from Malaysia’, IMF Working Paper, wp04129.
Dua, P., and Sen P., 2005. Delhi School of Economics, University of Delhi,
mimeo.
Froot, K., Thornton, D. (2002), ‘The Persistence of Emerging Market Equity’,
‘Emerging Markets Review’. December; 3(4): 338-64
Gordon, J., Gupta, P. (2003), ‘Portfolio Flows into India: Do Domestic
Fundamentals Matter?’ Working Paper No. 03/20, January.
Gupta, P. (2005), ‘Macroeconomic Determinants of Remittances: Evidence
from India’, IMF Working Paper, wp05224.
Johansen, S. (1988), ‘Statistical Analysis of Cointegrated Vector’, Journal of
Economic Dynamics and Control, 12, 231-54
Kim, E., Singal, V. (2000), ‘The Fear of Globalizing Capital Markets’, Emerging
Markets Review, November, 1(3): 183-98
Kohli, R, (2003), ‘Capital Flow and Domestic Financial Sector in India’,
Economic and Political Weekly, February.
Krugman, P. 1998. ‘What Happened to Asia?’ Manuscript. MIT. January.
Levine, R. (2001), ‘International Financial Liberalization and Economic
Growth’, Review of International Economics, November; 9(4): 688-702.
Lagoarde-Segot, T. and Lucey, B. (2006), ‘Financial Contagion in Emerging
Markets: Evidence from the Middle East and North Africa’, The Institute for
International Integration Studies Discussion Paper Series, iiisdp114, IIIS.
Mody, A., Murshid, A. (2005), ‘Growing Up with Capital Flows’, Journal of
International Economics, January, 65(1): 249-66.
Mohan, R. (2004), Fiscal Challenges of Population Aging: The Asian
Experience, speech at the symposium sponsored by the Federal Reserve Bank
of Kansas City, August 26 – 28.
Mohan, R. (2005), ‘Indian Economy in a Global Setting’, Address Delivered
at the Indian Merchants Chamber, Mumbai, September
Mohan, R. (2009), Capital Flows and Emerging Market Economies, CGFS
Papers No. 33, Bank for International Settlements, January.
Otker-Robe, I. Polanski, Z., Topf, B. and Vavra, D. (2007), ‘Coping With Capital
Inflows: Experiences of Selected European Countries’, IMF Working Paper
No. 07/190.
Pesaran M. and B. Pesaran (1997): Working with Microfit 4.0 Interactive
Economic Analysis, Oxford, Oxford University Press.
Pesaran, M., Y. Shin and R. Smith (1996): ‘Testing for the Existence of a Long-
Run Relationship’, Department of Applied Economics Working Paper No. 9622,
Cambridge University, also available on http://www.econ.cam.ac.uk/faculty/
pesaran/ (Accessed in January 2007).
Pesaran, M., Y. Shin and R. Smith (1999): ‘Bounds Testing Approaches to the
Analysis of Long-run Relationships’, Cambridge Working Papers in Economics,
No. 9907, University of Cambridge.
Pesaran, M., Y. Shin and R. Smith (2001): ‘Bounds Testing Approaches to the
Analysis of Level Relationships’, Journal of Applied Econometrics, Vol. 16,
No. 3, pp. 289-326.
Pesaran, H and Shin, Y (1998): ‘Generalised Impulse Response Analysis in
Linear ultivariate Models’, Economics Letters, Elsevier, Vol. 58(1), pp 17-29,
January.
Phillips, P. and P .Perron (1988), Testing for a Unit Root in Time Series
Regression, Biometrica, 75, 335-46
Rangarajan, C. (1993), Report of the High Level Committee on Balance of
Payments, April.
Rai, K. and Bhanumurthy, N. (2004), ‘Determinants of Foreign Institutional
Investment in India: The Role of Return, Risk and Inflation’, The Developing
Economies, XLII-4, December, 479–93
Reddy, Y.V. (1998), ‘Managing Capital Flows’, RBI Bulletin, December.
Reddy, Y.V. (1999), ‘External Debt Policies in Emerging Economies: New
Dimensions’, RBI Bulletin, July.
Reddy, Y.V. (2000), ‘Capital Flows and Self Reliance Redefined’, RBI Bulletin,
August.
Reserve Bank of India (2004a), Handbook of Statistics on Indian Economy,
RBI: Mumbai.
Reserve Bank of India (2004b), Report on Trend and Progress of Banking in
India (various years), RBI: Mumbai.
Ross, L. (2001), ‘International Financial Liberalization and Economic Growth’
Review of International Economics, vol. 9, issue 4, pages 688-702
Sarno, L., Taylor, M. (1999), ‘Moral Hazard, Asset Price Bubbles, Capital
Flows, and the East Asian Crisis: The First Tests’, Journal of International
Money and Finance, August, 18(4): 637-57.
Taylor, M. and Sarno, L. (1997), ‘Capital Flows to Developing Countries:
Long- and Short-Term Determinants’, World Bank Economic Review, 11 (3),
September, 451-70
Trivedi, P., and A. Nair (2003), “Determinants of FII Investment Inflow to
India.”, Paper presented at the Fifth Annual Conference on Money & Finance
in the Indian Economy, held by Indira Gandhi Institute of Development
Research, Mumbai, January 30–February 1.
World Bank (2007), ‘Managing Large Capital Inflows’, World Economic
Outlook, chapter 3.
Sinha, C. and Pradhan, N. C. (2008), ‘Regional Financial Integration in Asia:
Present and Future’, BIS Papers No. 42, October. |