by Shobhit Goel, Dirghau K. Raut, Madhuresh Kumar and Manu Sharma^ Artificial Intelligence (AI) is being increasingly adopted in the banking and financial services industry, with multiple use cases including fraud detection, customer segmentation, and chat automation. This article attempts to construct a quantitative measure of AI adoption in the Indian banking system using a text-mining approach. It also attempts to identify the impact of bank-specific characteristics in driving AI adoption using a panel fixed effects model. The results indicate that AI adoption is gaining momentum led by private banks, with asset size and CRAR influencing the rate of adoption. Introduction The banking and financial sector has a long and storied history of adapting to and adopting technological innovations, starting from the invention of paper currency in the 7th century AD to the introduction of the internet and mobile banking in the 21st century. In each case, the industry initially faced scepticism and challenges in embracing these new technologies, but eventually, they became integral to its functioning and productive efficiencies. Artificial Intelligence (AI) today can be broadly defined as the branch of computer science that aims to create machines and systems that can perform tasks which normally require human intelligence, such as reasoning, learning, decision-making, and natural language processing (Holden, 1991; McCarthy, 1960, 1989, 2007; Russell and Norvig, 2010). While terms like machine learning, deep learning, unsupervised learning, and neural networks are often used in conjunction with artificial intelligence, they can be viewed as subsets of the broad field of AI (Chart 1)1. The rapid rise in computing power combined with the rise of unstructured data has supported the development of machine learning and other AI systems. Further, the rise of smart devices powered by AI and the Internet of Things (IoT) has in turn created new swathes of data which can be used to create the next generation of AI solutions. Artificial intelligence (AI) and machine learning (ML) have gained prominence, especially with advancements in generative AI models based on large language models (LLMs). The adoption of AI and related technologies in the banking, financial services, and insurance (BFSI) sector is rapidly transforming the landscape of financial services both globally and in India (RBI, 2023). The banking sector is integrating AI into banking operations to enhance efficiency, accuracy, and customer experience, paving the way for a more innovative and customer-centric financial ecosystem (BIS, 2024).  The potential adoption and usage of the AI models has necessitated policy discussion and research around multiple macro financial and other issues including, quantitatively measuring the adoption of these technologies, identifying the factors driving their adoption, and evaluating the impact of these technologies. While there exists literature on the adoption of AI it is mostly qualitative in nature. This paper attempts to provide a quantitative measure of the pace of adoption of AI in Indian banks. It employs text-mining techniques to analyse 32 Indian commercial banks’ annual reports2 for eight years from FY 2015-16 to FY 2022-23 to identify their adoption and usage of AI and related technologies and their growth in recent years. It then explores the role of bank’s financial health in influencing AI adoption by utilising a fixed effects panel data model. The rest of the paper is divided into four sections. Section II provides stylised facts such as applications and business drivers of AI and its potential value creation in the banking sector. Data sources and methodology are explained in Section III while outcomes of text mining and empirical results on drivers of AI adoption are provided in Section IV. Section V concludes the article. II. Artificial Intelligence and the Financial Sector The digital age has witnessed a monumental shift in the pace and scale of innovation, with convergence of big data, advanced algorithms, and computational power. This rapid evolution of deep learning, machine learning, and other AI technologies has been accompanied by its increasing adoption and integration in the financial sector. While some view it as a natural evolution in this era marked by the banking sector and the broader financial industry’s relentless pursuit of efficiency, innovation, and enhanced decision-making capabilities, others have argued that the adoption of these technologies has become a necessity as the wider economy has embraced them. The banking industry is realising the potential of not only the traditional data analytics and machine learning but it is increasingly recognising the role of new and emerging advanced AI technologies like deep neural networks and large language models to derive value in traditional areas like marketing and sales, risk management, finance, and IT. According to McKinskey (2021), the potential for value creation through AI and related technologies in global banking is more than US$1 trillion annually mainly in the domain of marketing and sales, and risk management (Chart 2). From the broader business drivers’ standpoint, within the Indian financial sector, the improvement of customer experience, revenue augmentation, and the creation of new products – all of which can be broadly seen as endeavours in the realm of marketing and sales – stand out as significant motivation for the utilisation of AI (Chart 3a). Indian banks are increasingly focusing on deployment of AI-driven chatbots and virtual assistants to enhance customer experience and provide personalised service (Chart 3b) (PwC India and FICCI, 2022). These technologies provide customers with instant responses to queries, streamline account management, and offer personalised financial advice (Alt et al., 2021; Boukherouaa et al., 2021; Goudarzi et al., 2018; Orçun Kaya, 2019). AI technologies are reshaping traditional banking and expanding financial services to the underserved populations. Mobile banking apps, AI-driven credit scoring, and blockchain-based solutions are examples of some of the powerful and enabling vehicles of greater financial inclusion by extending access to banking services to previously unbanked or underbanked individuals (Bazarbash, 2019; Gensler and Bailey, 2021). 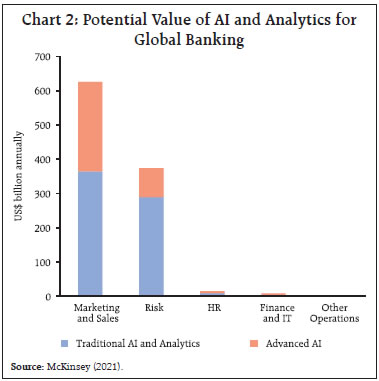 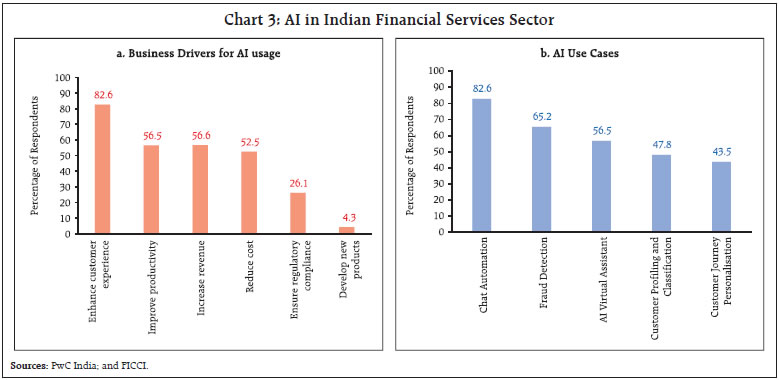 Banks and FIs are using Machine Learning (ML) algorithms as part of their proprietary trading desks, forex trading desks and even fixed income trading to deploy sophisticated trading strategies on a real-time basis, with the goal of increasing the profitability of their trading operations. These strategies are increasingly driven by predictive analytics, sentiment analysis, and other AI-powered tools, with the potential to outperform traditional investment approaches (Boukherouaa et al., 2021; Goudarzi et al., 2018). Some of the areas for which banks are using AI to save cost are: conversational banking, anti-fraud and under-writing3. Financial sector in India is also trying to leverage the AI tools for regulatory compliance. AI solutions could facilitate compliance by automating reporting, monitoring transactions for suspicious activities, better understanding of the regulatory requirements and ensuring adherence to evolving regulatory standards, reducing the risk of regulatory misconducts, penalties and fines (Boukherouaa et al., 2021). As seen earlier, AI solutions have huge potential to enhance risk management capabilities, by undertaking analysis of massive datasets, finding solutions not available earlier and getting better perspectives on the real-time market conditions; thereby allowing for more accurate and timely business risk predictions, contributing to improved portfolio management, loan underwriting, and fraud detection. According to a survey of the IT executives in the banking sector globally, banks are deploying AI based applications for risk management, the most prominent of which are for fraud detection, optimising IT operations, risk assessment and personalising credit scoring (Chart 4). 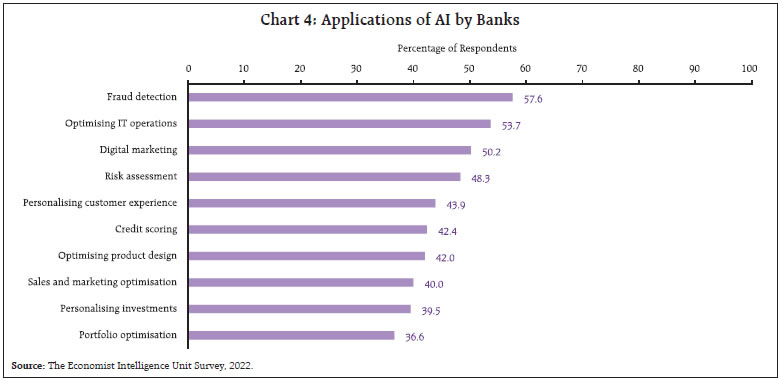 AI-powered fraud detection systems can identify suspicious activities and transactions in real-time, thus offering a robust defense against financial crimes. Some experts argue that future of fraud detection has to be AI powered as financial transactions are increasingly occurring in the digital realm, making the battle against fraud more complex (Boukherouaa et al., 2021; Goudarzi et al., 2018; Milojević and Redzepagic, 2021; Orçun Kaya, 2019). With the growing volume of sensitive financial data, cybersecurity has become a paramount concern. Banks and FIs are increasingly adopting AI-powered cybersecurity tools for automated anomaly detection and predictive behavioural analysis to identify potential threats and vulnerabilities (Aziz and Dowling, 2018; Donepudi, 2017; Goudarzi et al., 2018; Milojević and Redzepagic, 2021; Orçun Kaya, 2019). III. Data Sources and Methodology Most of the existing literature on the impact of AI/ML on financial sector has limited quantitative information on the actual usage and adoption of artificial intelligence, machine learning and other automation technologies in the Indian banking sector. The existing literature has mainly adopted three broad approaches. The first approach has been a completely qualitative one, where analysis of academic journals, industry reports and leading business publications is undertaken to identify use cases of AI in the industry. However, the main shortcomings of this approach are: (1) Possibility of researchers’ bias which can lead to an overemphasis on certain themes or findings, and underrepresentation of others; (2) Concerns over rigour and reliability of reports; (3) Limited ability to generalise findings and allow spatial and inter-temporal comparisons (Carter et al., 2021; Rahman, 2016). The second approach has been to conduct surveys/questionnaires and provide qualitative overview of AI adoption or convert the responses into a quantitative metric. However, this approach too suffers from issues like (1) difficulty in capturing the complexity with adoption of a frontier technology like AI; (2) unreliability and bias as the respondent may not be aware of all aspects or use cases of technology within the firm and may also overstate and over emphasise their usage of AI to appear more innovative or may understate the response due to privacy concerns; (3) Comparison issues especially across time which is a very important aspect as technology adoption is a dynamic process and a firm’s use of AI and other technologies can evolve rapidly (Carter et al., 2021; Survey Research and Questionnaires, n.d.).The third approach has been to use a proxy variable as a measure of AI adoption including using some proportion of IT investment expenditure/capital expenditure/M&A expenditure of technology related firms. The main shortcomings of this approach are (1) these metrics measure not just AI based technologies but also other types of IT expenditure (2) expenditure may not directly lead to adoption as firms may fail to successfully develop or operationalise a technology solution or may be utilising cost-effective open source technologies to develop solutions. In view of this, we propose a text mining-based approach to measure the adoption of AI/ML and related technologies in the Indian banking sector. We perform text mining on the annual reports of the Indian banks as they are highly likely to reflect the usage and adoption of new-age technology like AI/ML. Further, the annual reports are a trustworthy and publicly available source of information and are also available across years thereby allowing for inter-temporal analysis. We have adopted a dictionary-based approach after parsing the annual reports to extract individual words/phrases and evaluate the instances of usage of these words. While the earliest work utilised existing dictionaries like Harvard word dictionary, subsequent work created their own unique dictionaries according to the specific need. These text mining-based approaches have been used to measure otherwise hard to evaluate attributes like corporate governance, ESG activity and FinTech adoption (Table 1). Table 1: Literature Utilising Text Analytics | Loughran et al. (2009) | Undertook text mining to examine the occurrence of ethics-related terms in firms’ 10-K reports. Found that firms using ethics-related terms are more likely to be (i) "sin" stocks, (ii) the object of class action lawsuits and (iii) to score poorly on measures of corporate governance. | Shirata et al. (2011) | Adopted a text mining approach to analyse Japanese firms’ financial reports and extracted key phrases/descriptions which could be able to predict bankruptcy. | Feng et al. (2013) | Undertook textual analysis of firms 10-K reports to create a word count based measure of industry competition. This measure is related to existing industry-level measures of disclosure (e.g., Herfindahl index), but provide additional insight into both across-industry variation and within-industry variation. | Baier et al. (2020) | Undertook textual analysis of firms’ 10-K reports for measuring environmental, social and governance (ESG) activity of the firms. Undertook a word count based measure using a uniquely created dictionary for ESG related words. | Hong et al. (2023) | Constructed a new measure of FinTech adoption by firms, using a word count approach with a uniquely created dictionary for FinTech on firms’ 10-K reports. Further, examined how product market competition affects FinTech adoption at the firm level and found that firms operating in more competitive environments have a higher incentive to adopt FinTech. | Sharma et al. (2024) | Undertook natural language processing (NLP) based text mining approach to analyse news articles, speeches, interviews and other publicly available documents for central banks and multi-lateral institutions. They further attempt to decipher the policy focus and priority of the central banks and multilateral institutions in the areas of FinTech and the shift in these trends over time. | The key step in performing a keyword and named entity matching is using a suitable dictionary which contains a list of words or phrases related to specific categories or concepts of interest. In line with existing literature, we started by using the popular dictionaries/glossary related to AI and ML including those by Google Vertex AI4, Google Developers5, IBM6, NHS AI Lab7 and Council of Europe8 (RBI, 2023). We utilise the popular LLM models of ChatGPT and Bard to create a dictionary of AI related terms pertaining to financial sector. The advantage of using LLM models to create a dictionary comes from the fact that LLM models have been trained on an extensive dataset and therefore better equipped to identify keywords related to AI in the context of financial sector. A comprehensive dictionary has been formulated by combining all these dictionaries. We have employed a combination approach for creating the dictionary where both keyword matching and named entity recognition are used. This approach is based on searching for presence of AI related keywords such as "artificial intelligence," "machine learning," "neural networks," "deep learning," "data science," and other relevant terms. We also match for named entities related to AI, like AI applications (e.g., natural language processing, computer vision), or AI-related organisations (e.g., ChatGPT). A higher frequency of AI-related keywords indicates a higher likelihood of deployment or focus on AI. Words which may be used in an alternate usage sense in financial sector have been removed along with terms which were found to have negligible mention in the test case to improve accuracy and parsimony gains, respectively. As is the case with any empirical methodology, this methodology also has certain limitations which need to be acknowledged9. The quantitative mentions of AI-related terms in the dictionary-based frequency measures may not account for the contextual meaning (Loughran and Mcdonald, 2016). The presence of AI related keywords may be indicating discussion about AI related opportunities and risks rather than its deployment (Chen et al., 2023). On the other hand, it may fail to account for banks that have implemented AI extensively but have not emphasised it as frequently in their reports, leading to underestimation of AI adoption. Thus, the methodology captures banks discussion and focus on AI and related technologies (which could be the part of their preparedness/evaluation of these technologies before its deployment) and not just the deployment of these technologies. Annual reports for 32 commercial banks10 11 for 8 years, starting from FY 2015-16 to FY 2022-23, were analysed. In the first step, the annual reports of the said banks were downloaded in portable document format (pdf). In the text pre-processing stage, these pdf files were checked for machine readability and made machine readable, wherever possible, using techniques like optical character recognition (OCR). The machine-readable pdfs were then processed individually, by extracting the text content from each page and storing it in a data-frame. In the next step, tasks such as lowercasing, removing special characters, tokenisation stop-word removal, and lemmatisation were performed to make the text suitable for dictionary matching. In the last step, keyword matching was done by checking each keyword for its presence in the text data, and the frequency of occurrence was recorded. The key banking characteristics including total assets, CRAR (capital to risk weighted assets ratio), return to total assets, efficiency ratio, GNPA (gross NPAs to gross advances) ratio, NNPA (net NPAs to net advances) ratio and retail lending ratio (gross retail loans to gross advances) for both public and private sector banks have been outlined in Table 2. We find that there are considerable differences at aggregate level between private and public sectors in terms of these banking characteristics. Table 2: Key Banking Characteristics of Public and Private Sector Banks in India | Year | Total Assets (in ₹ lakh crore) | Capital Ratio (CRAR) | Return on Total Assets (annualised) | Efficiency (Cost to Income Ratio) | Gross NPAs to Gross Advances (Per cent) | Net NPAs to Net Advances (Per cent) | Retail Lending Ratio (Per cent) | | Public Sector Banks | 2015-16 | 81.01 | 11.82 | -0.27 | 53.02 | 9.83 | 6.13 | 17.4 | 2016-17 | 88.15 | 12.14 | -0.16 | 50.26 | 12.47 | 7.39 | 19.6 | 2017-18 | 91.79 | 11.66 | -0.94 | 52.32 | 15.52 | 8.58 | 22.4 | 2018-19 | 93.73 | 12.20 | -0.77 | 55.18 | 12.25 | 5.12 | 23.4 | 2019-20 | 99.88 | 12.85 | -0.29 | 53.33 | 10.79 | 4.00 | 25.0 | 2020-21 | 109.13 | 14.04 | 0.29 | 51.46 | 9.36 | 3.23 | 26.8 | 2021-22 | 119.87 | 14.62 | 0.55 | 53.09 | 7.57 | 2.33 | 27.8 | 2022-23 | 130.39 | 15.53 | 0.75 | 51.77 | 5.22 | 1.34 | 29.2 | | Private Sector Bank | 2015-16 | 28.91 | 15.68 | 1.64 | 44.11 | 2.70 | 1.27 | 24.8 | 2016-17 | 34.39 | 15.53 | 1.45 | 43.19 | 3.51 | 1.84 | 25.5 | 2017-18 | 41.17 | 16.43 | 1.27 | 43.83 | 4.01 | 1.97 | 25.4 | 2018-19 | 51.17 | 16.07 | 0.82 | 46.60 | 4.81 | 1.89 | 26.8 | 2019-20 | 56.77 | 16.55 | 0.51 | 43.66 | 5.11 | 1.43 | 30.2 | 2020-21 | 63.45 | 18.42 | 1.22 | 41.50 | 4.74 | 1.41 | 31.7 | 2021-22 | 72.40 | 18.78 | 1.44 | 44.83 | 3.73 | 0.96 | 30.5 | 2022-23 | 83.01 | 18.61 | 1.60 | 48.38 | 2.17 | 0.55 | 35.2 | Source: Database on India Economy, RBI. | IV. Results IV.1 Artificial Intelligence Adoption The results show that initially (FY 2015-16), public sector banks were proactively considering AI/ML and related technologies, showing a similar mention of AI related keywords (AI score) compared to their private sector counterparts. However, during the period from 2016 to 2021, the AI-related word count in the annual reports of private sector banks has picked up. This may be due to a combination of recognition of additional use cases of existing AI based technologies along with more agility in adopting the newer and advanced AI techniques and models. The usage of AI related keywords in the annual reports of private sector banks increased by approximately six-fold in 2022-23 reports as compared to 2015-16 level. Even in case of public sector banks (PSBs), the emphasis on new age technologies like AI in their annual reports has increased more than 3 times between 2015-16 and 2022-23 (Chart 5). In a few public-sector banks, the enthusiasm towards AI based technologies is broadly at par with their private sector peers, especially in recent years.  Further, the word cloud reveals interesting insights with most banks focussing on automation which may be due to a push for efficiency gains and reduce human interventions (Chart 6). Data analytics is another major thrust area with possible usage in fraud detection and predictive analytics. While cloud computing and big data continue to be the major technologies which banks are employing, there is an increasing recognition of potential usage of the newer AI and ML technologies like Robotic Process Automation (RPA), Internet of Things (IoT) and Natural Language Processing (NLP). IV.2 Drivers of AI Adoption A panel fixed effect model was used to evaluate how the AI score, as measured by text analytics (explained above in section IV.1), may be influenced by bank-level characteristics like assets, capital to risk weighted assets ratio (CRAR), return on assets (RoA) and gross non-performing assets (GNPA). The data on bank specific variables has been taken from RBI’s Database on Indian Economy. The choice of a fixed effects model was made as the time-invariant unobserved characteristics of each bank could influence both the AI adoption score and the bank-level characteristics. For instance, a bank’s management quality or corporate culture could influence its AI adoption score. By using a fixed effects model, we can control for these unobserved characteristics and isolate the relationship between AI adoption and the observed bank-level characteristics. Before estimating the model, we performed two diagnostic tests: the Hausman test and the Redundant Fixed Effects test. The Hausman test is used to decide between a fixed effects model and a random effects model. The null hypothesis under this test is that the preferred model is random effects. However, in our case, we rejected the null hypothesis, suggesting that the fixed effects model is more appropriate. The Redundant Fixed Effects test checks whether the fixed effects (or individual-specific effects) in the model are significant. The null hypothesis of this test is that these effects are redundant. Again, we rejected the null hypothesis, indicating that these fixed effects are significant and should be included in our model. Estimation results of panel fixed models are provided in Table 3. In the baseline model (Model 1), AI score is regressed upon assets, capital position and dummies representing merger year and private-sector banks. In the subsequent estimation, we augment our model to include other banking indicators such as GNPA (Model 2), RoA (Model 3), and efficiency ratio (Model 4) and retail lending ratio (Model 5). The specification for the fully augmented model (Model 5) is as follows: 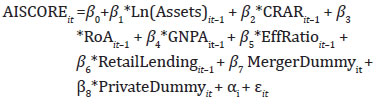 In this equation AISCOREit is the dependentvariable. Ln(Assets)it–1 is the natural log of assets of a bank (in crores of rupees)12 and has been mean-centered to account for the scale issue. Asset size can be viewed as proxy of size of banks and it can potentially influence adoption of technologies like AI as it affects the amount of resources available, risk taking capacity, exploitation of economies of scale, potential benefits and even executional challenges (Bordonaba-Juste et al., 2012; Burke, 2005; Hall and Khan, 2003; Lee and Xia, 2006; Na et al., 2023). CRARit–1 is capital to risk-weighted assets ratio and measures the capital adequacy, thus a proxy for the financial health of the banks which in turn indicates availability of capital to afford upfront costs of acquiring new technologies which can be significant in case of complex and capital intensive technologies like AI (Chhaidar et al., 2023; Hall and Khan, 2003; Nugroho et al., 2017). RoAit–1 is return on assets expressed in percentage which is a measure of how productively the bank has been able to utilise its assets and thus a measure of its capital efficiency in general (Adhitya and Sembel, 2020; Chhaidar et al., 2023; Doran et al., 2022). GNPAit–1 stands for gross non-performing assets. Banks with higher GNPA may derive greater benefits from utilising AI related technologies in credit underwriting and recovery process (Bazarbash, 2019; Chhaidar et al., 2023; Doran et al., 2022; Goudarzi et al., 2018; Seth and Bhavika Gandhi, 2023). However, banks with higher GNPAs could be more focussed on cleaning books and also have had to allocate more resources toward provisioning needs, thus reducing the resources available for investing in AI and related technologies. In model 2a, net non-performing assets (NNPA) has been taken instead of GNPA as an alternate specification. EffRatioit–1 is efficiency ratio which represents the cost to income ratio for a bank. Efficiency ratio can be indirect proxy for measuring the potential benefits of using AI related technologies to reduce costs (Alt et al., 2021; Boukherouaa et al., 2021; Orçun Kaya, 2019). RetailLendingit–1 is the retail lending ratio which represents how retail centric is a bank. With a lot of AI technologies focussed towards improving the retail customer experience like creation of chatbots, wealth management solutions, recommendation models among others. It is possible that banks which have higher proportion of retail loans are more retail banking focussed and thus have higher adoption of AI and related technologies. All the bank specific variables have been taken in lag form to account for the possibility that the impact of these variables on the AI adoption score may not be immediate, but rather delayed. Moreover, using lagged independent variables can also help mitigate potentialendogeneity problems. MergerDummyit takes value 1 for the bank in the year it has been merged and 0 otherwise, thus allows us to estimate the short-term impact of the merger13. PrivateDummyit is the dummy variable taking value 1 for private sector banks and 0 otherwise, which allows to control for potential differences between these two categories. αi represents the individual-specific (bank-specific) effects and εit is the error term. The subscript i represents the cross-section (i.e. bank) and t represents the time period. We find consistent results across all the models considered (Table 3). Across all models, we find that AI score is positively related to the asset size of the banks as evident from positive and statistically significant coefficient, suggesting higher adoption by larger banks. This finding aligns with resource-based theory, which posits that organisations with greater resources are more inclined to invest in innovation and modern technologies like AI (Barney, 1991) and survey results showing higher AI adoption rate among banks having larger asset size14. Further, larger banks owing to their difficulties in coordination across verticals are likely to achieve higher net gains from adoption of such technologies and data integration, thereby increasing the motivation for adoption of AI. It may also be indicating that the adoption of technologies such as AI is relatively difficult for smaller banks due to larger fixed cost and absence of economies of scale. Table 3: Factors Impacting Adoption of AI in Indian Banks | | Model 1 | Model 2 | Model 2a | Model 3 | Model 4 | Model 5 | Ln_assets | 49.34*** | 48.62*** | 49.09*** | 48.40*** | 48.72*** | 50.13*** | CRAR | 1.68*** | 1.78*** | 1.90*** | 1.88*** | 1.98*** | 1.98*** | GNPA ratio | | 0.31 | | 0.19 | 0.25 | 0.26 | RoA | | | | -0.79 | -0.04 | 0.01 | Efficiency ratio | | | | | 0.17 | 0.20 | Retail Lending ratio | | | | | | -0.10 | Merger dummy | -6.58 | -6.70 | -6.40 | -6.70 | -6.19 | -6.50 | Private dummy | 20.51* | 17.05 | 21.56* | 17.23 | 17.06 | 18.14 | NNPA ratio | | | 0.62 | | | | Cross-Sections Included | 32 | 32 | 32 | 32 | 32 | 32 | Total Observations | 256 | 256 | 256 | 256 | 256 | 256 | Constant | Included | Included | Included | Included | Included | Included | R2 | 0.76 | 0.76 | 0.76 | 0.76 | 0.76 | 0.76 | Adjusted R2 | 0.72 | 0.72 | 0.72 | 0.72 | 0.72 | 0.72 | F-statistic | 19.49*** | 18.98*** | 19.03*** | 18.41*** | 18.05*** | 17.54*** | Akaike info | 8.39 | 8.39 | 8.39 | 8.40 | 8.40 | 8.40 | Schwarz | 8.88 | 8.90 | 8.90 | 8.92 | 8.94 | 8.96 | Hannan-Quinn | 8.58 | 8.60 | 8.60 | 8.61 | 8.61 | 8.62 | Durbin-Watson | 1.24 | 1.23 | 1.24 | 1.24 | 1.28 | 1.29 | ***, ** and * denotes statistical significance at 1 per cent, 5 per cent and 10 per cent probability level, respectively. | Capital-to-risk weighted asset ratio (CRAR) which is a proxy for the capital adequacy of the bank and thus a reflection of the financial health of the bank, is positively related to AI score. This result resonates with the viewpoint that well-capitalized banks are better positioned to take the investment risks in new technology in terms of adequate capital buffers and confidence to pursue AI solutions. Other bank specific indicators such as RoA, GNPA/NNPA, efficiency ratio and retail lending are not statistically significant for explaining AI score15. The merger dummy is also not found to be a statistically significant determinant of AI score of banks. The coefficient for the dummy variable representing private sector banks though positive is only significant at 10 per cent level in Model 1 and 2a. This result needs to be seen in light of analytical findings where both private and public-sector banks broadly started at similar level of AI score in 2015-16 but then saw private sector banks AI score increase rapidly in the next 5 years. However, in the last 2 years, public sector banks also appear to have enhanced their AI adoption, as observed in their AI score, possibly reflecting the broad-based ongoing expansion of digital technologies in the Indian banking sector. The greater adoption of AI in private sector banks could be due to a larger proportion of their clientele being better equipped to access digital services and more comfortable with usage of modern technology-based solution (ET BFSI, 2021; Malladi et al., 2021). Also, private banks often cater to more financially aware and affluent customers and therefore could see higher potential for leveraging AI based solutions like customer segmentation, robo-advisory, robo-wealth management tools to cross-sell or provide other financial services. Further, private sector banks especially those with a smaller branch network, are much more likely to adopt AI based solutions to gain new customers or cross-sell different products, as it represents a more cost-effective solution. On the other hand, PSBs already have well established offline channels, especially in rural and semi-urban areas. However, with the rapid advancements in AI, especially generative AI and LLM based models in last 2 years, which have been accompanied with public at large being able to access and thus subsequently draw comfort with AI based solutions, public sector banks also appear to be increasing their usage of AI based solutions. V. Conclusion While AI is being increasingly explored in every industry, its usage is expected to have a profound impact on various operations of banking and finance, including risk assessment, fraud detection, customer service, investment strategies, regulatory compliance, and more. As the capabilities of these technologies continue to grow, so does their influence on the decision-making processes. AI is expected to have the potential to reduce the inefficiencies, through automation by minimising errors in the human decision making and by providing cost effective solutions. It is also expected to make banking services accessible to the population at the bottom of the pyramid. While the integration of AI into banking and finance offers immense opportunities, it also presents challenges such as possibility of bias, lack of transparency, and issues surrounding the ethical use of data which requires an in-depth evaluation in view of its implications for financial sector and the overall economy. The recent policy initiatives by India including the National Strategy for Artificial Intelligence, IndiaAI Mission, AI for India 2.0 and Skill India AI Portal aim to reap the potential offered by AI and related technologies while being cognisance of the risks and challenges presented by it. The Reserve Bank of India has also recognised the potential of AI/ML and related technologies and encouraged the banks to appropriately adopt these technologies for conducting ongoing due diligence and effective monitoring for KYC/AML norms16. Text mining of annual reports of Indian banks during 2015-16 to 2022-23 reveals that both private and public-sector banks are increasingly emphasising on AI and related technologies; however, the pace of increase is higher for private banks. Automation, data analytics, cloud computing and big data are the major thrust areas, with increasing consideration for RPA, IoT and NLP like technologies by banks especially in recent years. Among different banking indicators, size and financial health of a bank is found to positively influence the bank’s focus on AI, reflecting the impact of economies of scale and the availability of investment on the technological advancement. References Adhitya, A., and Sembel, H. M. R. (2020). the Impacts of Mobile Banking Technology Adoption on the Financial Performance and Stock Performance of Big Banks in Indonesia. South East Asia Journal of Contemporary Business, Economics and Law, 22(1), 63–73. Alt, M.-A., Vizeli, I., and Săplăcan, Z. (2021). Banking with a Chatbot – A Study on Technology Acceptance. Studia Universitatis Babes-Bolyai Oeconomica, 66(1), 13–35. https://doi.org/10.2478/subboec-2021-0002 Aziz, S., and Dowling, M. M. (2018). AI and Machine Learning for Risk Management. SSRN Electronic Journal. https://doi.org/10.2139/ssrn.3201337 Baier, P., Berninger, M., and Kiesel, F. (2020). Environmental, social and governance reporting in annual reports: A textual analysis. Financial Markets, Institutions and Instruments, 29(3), 93–118. https://doi.org/10.1111/fmii.12132 Barney, J. (1991). Firm Resources and Sustained Competitive Advantage. Journal of Management, 17(1), 99–120. Bazarbash, M. (2019). FinTech in Financial Inclusion Machine Learning Applications in Assessing Credit Risk (WP/19/109). BIS. (2024). Digitalisation of finance (Issue May). www.bis.org Bordonaba-Juste, V., Lucia-Palacios, L., and Polo-Redondo, Y. (2012). The influence of organizational factors on e-business use: Analysis of firm size. Marketing Intelligence and Planning, 30(2), 212–229. https://doi.org/10.1108/02634501211211984/FULL/XML Boukherouaa, E. B., Shabsigh, G., Deodoro, J., Farias, A., Iskender, E. S., Mirestean, A. T., and Ravikumar, R. (2021). Powering the Digital Economy: Opportunities and Risks of Artificial Intelligence in Finance. https://www.imf.org/en/Publications/Departmental-Papers-Policy-Papers/Issues/2021/10/21/Powering-the-Digital-Economy-Opportunities-and-Risks-of-Artificial-Intelligence-in-Finance-494717 Burke, K. (2005). The Impact of Firm Size on Internet Use in Small Businesses. Electronic Markets, 15(2), 79–93. https://doi.org/10.1080/10196780500083738 Carter, S. M., Shih, P., Williams, J., Degeling, C., and Mooney-Somers, J. (2021). Conducting Qualitative Research Online: Challenges and Solutions. Patient, 14(6), 711–718. https://doi.org/10.1007/s40271-021-00528-w Chen, J., Henry, E., and Jiang, X. (2023). Is Cybersecurity Risk Factor Disclosure Informative? Evidence from Disclosures Following a Data Breach. Journal of Business Ethics, 187(1), 199–224. https://doi.org/10.1007/s10551-022-05107-z Chhaidar, A., Abdelhedi, M., and Abdelkafi, I. (2023). The Effect of Financial Technology Investment Level on European Banks’ Profitability. Journal of the Knowledge Economy, 14(3), 2959–2981. https://doi.org/10.1007/s13132-022-00992-1 Donepudi, P. K. (2017). Machine Learning and Artificial Intelligence in Banking. Engineering International, 5(2). Doran, N. M., Bãdîrcea, R. M., and Manta, A. G. (2022). Digitization and Financial Performance of Banking Sectors Facing COVID-19 Challenges in Central and Eastern European Countries. Electronics (Switzerland), 11(21). https://doi.org/10.3390/electronics11213483 ET BFSI. (2021). Public Sector Bank: How PSU banks are catching up in the digital world. Economic Times. https://bfsi.economictimes.indiatimes.com/news/banking/how-psu-banks-are-catching-up-in-the-digital-world/84541392 Feng, L., Lundholm, R., and Michael, M. (2013). A measure of competition based on 10-k filings. Journal of Accounting Research, 51(2), 399–436. https://doi.org/10.1111/j.1475-679X.2012.00472.x Gensler, G., and Bailey, L. (2021). Deep Learning and Financial Regulation. SSRN Electronic Journal, 1–45. https://doi.org/10.2139/ssrn.3788662 Goudarzi, S., Hickok, E., and Sinha, A. (2018). AI in Banking and Finance. In The Centre For Internet and Society. Hall, B. H., and Khan, B. (2003). Adoption of a New Technology (No. 9730; Issue May). https://doi.org/10.4018/978-1-5225-7086-8.ch001 Holden, C. (1991). Machines Who Think (Vol. 254, Issue 5036). A K Peters. https://doi.org/10.1126/science.254.5036.1291-b Hong, L., Nikbakht, E., and Zhou, T. (2023). Does product market competition affect the adoption of FinTech by non-financial firms? Finance Research Letters, 54(August 2022), 103730. https://doi.org/10.1016/j.frl.2023.103730 Lee, G., and Xia, W. (2006). Organizational size and IT innovation adoption: A meta-analysis. Information and Management, 43(8), 975–985. https://doi.org/10.1016/J.IM.2006.09.003 Loughran, T., and Mcdonald, B. (2016). Textual Analysis in Accounting and Finance: A Survey. Journal of Accounting Research, 54(4), 1187–1230. https://doi.org/10.1111/1475-679X.12123 Loughran, T., Mcdonald, B., Yun, H., Loughran, T., and Yun, H. (2009). A Wolf in Sheep’s Clothing: The Use of Ethics-Related Terms in 10-K Reports. 89(2009), 39–49. https://www3.nd.edu/~tloughra/WolfSheep.pdf Malladi, C. M., Soni, R. K., and Srinivasan, S. (2021). Digital financial inclusion: next frontiers—challenges and opportunities. CSI Transactions on ICT, 9(2), 127–134. https://doi.org/10.1007/s40012-021-00328-5 McCarthy, J. (1960). Programs with common sense. McCarthy, J. (1989). Artificial Intelligence, Logic and Formalizing Common Sense. In Philosophical Logic and Artificial Intelligence (pp. 161–190). Springer Netherlands. https://doi.org/10.1007/978-94-009-2448-2_6 McCarthy, J. (2007). From here to human-level AI. Artificial Intelligence, 171(18), 1174–1182. https://doi.org/10.1016/j.artint.2007.10.009 Milojević, N., and Redzepagic, S. (2021). Prospects of Artificial Intelligence and Machine Learning Application in Banking Risk Management. Journal of Central Banking Theory and Practice, 10(3), 41–57. https://doi.org/10.2478/jcbtp-2021-0023 Na, S., Heo, S., Choi, W., Han, S., and Kim, C. (2023). Firm Size and Artificial Intelligence (AI)-Based Technology Adoption: The Role of Corporate Size in South Korean Construction Companies. Buildings, 13(4). https://doi.org/10.3390/buildings13041066 Nugroho, M. A., Susilo, A. Z., Fajar, M. A., and Rahmawati, D. (2017). Exploratory Study of SMEs Technology Adoption Readiness Factors. Procedia Computer Science, 124, 329–336. https://doi.org/10.1016/j.procs.2017.12.162 Orçun Kaya. (2019). Artificial Intelligence in Banking Industry. Deutsche Bank Research. https://doi.org/10.1002/9781119710301.ch19 PwC India, and FICCI. (2022). Uncovering the ground truth: AI in Indian financial services. February, 1–36. Rahman, M. S. (2016). The Advantages and Disadvantages of Using Qualitative and Quantitative Approaches and Methods in Language "Testing and Assessment" Research: A Literature Review. Journal of Education and Learning, 6(1), 102. https://doi.org/10.5539/jel.v6n1p102 RBI. (2023). Report on Trend and Progress of Banking in India 2022-23. Russell, S. J., and Norvig, P. (2010). Artificial Intelligence: A Modern Approach (Vol. 4). Prentice Hall. https://doi.org/10.1109/ICCAE.2010.5451578 Seth, S., and Bhavika Gandhi. (2023). Revolutionizing risk assessment and lending decisions: The impact of digitalization and data analytics in Indian banks. In Advancements in commerce, economics and management: A research compilation (Issue September, pp. 111–118). Sharma, M., Raut, D. K., Goel, S., and Kumar, M. (2024). Evolution of FinTech and Central Banks : A Text Mining- Based Survey. RBI Bulletin, LXXVIII(August), 131–148. Shirata, C. Y., Takeuchi, H., Ogino, S., and Watanabe, H. (2011). Extracting key phrases as predictors of corporate bankruptcy: Empirical analysis of annual reports by text mining. Journal of Emerging Technologies in Accounting, 8(1), 31–44. https://doi.org/10.2308/jeta-10182 Survey Research and Questionnaires. (n.d.). Research Connections. Retrieved November 3, 2023, from https://researchconnections.org/research-tools/data-collection/survey-research-and-questionnaires The Economist Intelligence Unit. (2022). Banking on a game-changer: AI in financial services. The Economist, 1–9. Annexure Annex 1: Glossary of Major AI Related Terms | Term | Definition | Algorithm | A set of rules that a machine can follow to learn how to do a task. | Artificial Intelligence (AI) | AI refers to the simulation of human intelligence processes by machines, especially computer systems to perform cognitive tasks like thinking, perceiving, learning, problem solving and decision making. AI can have ‘communication’ or ‘decisions making’ similar to human. | Big data | Datasets that are too large or complex which may be structured, semi-structured and unstructured data that can be mined for information and used in machine learning projects, predictive modelling and other advanced analytics applications. | Chatbot | Chatbot is a program designed for communicating like humans with users/people through text or voice command. | ChatGPT | ChatGPT stands for ‘Chat Generative Pre-Trained Transformer’, which is a large-language-model based AI chatbot that uses natural language processing to create humanlike conversational dialogue. The language model can respond to questions and compose various written content, including articles, social media posts, essays, code and emails. | Computer Vision | An interdisciplinary scientific field that deals with how computers can gain high-level understanding from digital images or videos. | Data Mining | The process of discovering patterns in large data sets involving methods at the intersection of machine learning, statistics, and database systems. | Data Analytics | Data analytics is the science of analyzing raw data through tools, technologies, and processes. It is used to summarise data and to find trends pattern or to identify anomaly to improve decision-making, and foster business growth. Some types of data analytics such as prescriptive analytics and cognitive analytics are associated with AI/ML models such as predictive modelling, deep learning and natural language processing. | Data Science | An interdisciplinary field that uses scientific methods, processes, algorithms and systems to extract knowledge and insights from structured and unstructured data. | Deep Learning | A subset of machine learning which is based on artificial neural networks that has networks capable of learning complex patterns and relationships within data. Deep learning is a branch of machine learning. It is capable of learning. | Internet of Things (IoT) | It is a network of devices facilitating communication such as exchanging data with other devices/clouds and systems over the internet. | Machine Learning (ML) | Machine Learning is a type of artificial intelligence (AI) that allows software applications to become more accurate at predicting outcomes without being explicitly programmed to do so. It involves the use of algorithms to parse data and learn from it and making a determination or prediction. The machine gets "trained" using large amounts of data and algorithms, and in turn gains the capability to perform specific tasks. | Natural Language Processing (NLP) | A subfield of linguistics, computer science, and artificial intelligence concerned with the interactions between computers and human language. It is the ability of computers to understand text and spoken words like human being. | Neural Network | A neural network is an adaptive system that learns by using interconnected nodes or neurons in a layered structure that resembles a human brain. A neural network can learn from data, so it can be trained to recognize patterns, classify data, and forecast future events. | Predictive Analysis | The use of data, statistical algorithms and machine learning techniques to identify the likelihood of future outcomes based on historical data. | Robotic Process Automation (RPA) | The use of software with artificial intelligence (AI) and machine learning capabilities to handle high-volume, repeatable tasks that previously required humans to perform. | Sentiment Analysis | The use of natural language processing, text analysis, computational linguistics, and biometrics to systematically identify, extract, quantify, and study affective states and subjective information. | Supervised Learning | A type of machine learning where the model is provided with labeled training data. | Text Mining | Text mining is the process of extracting valuable information from unstructured text data to analyze, understand, and derive insights. It uses techniques of natural language processing and machine learning. | Unsupervised Learning | A type of machine learning where the model is not provided with labeled training data. | |