Press Release RBI Working Paper Series No. 03 A Measure of Finance-Neutral Output Gap for India @Deba Prasad Rath, Pratik Mitra and Joice John Abstract 1In the Indian context, the present literature on estimation of potential output - the level of output that an economy can produce sustainably over the medium term - is based on an understanding that sustainability is characterised as output consistent with low and stable inflation. However, there are episodes when economic growth has been on an unsustainable path in spite of stable inflation suggesting that there could be other sources instilling imbalance into the system. Informed by the recent literature which has recognized the key role played by the financial variables in explaining the output gap, this paper suggests that inclusion of information from the financial sector yields measures of output gaps that are significantly different from the traditional output gap estimates, especially in case of recent period which demonstrates higher slack in the economy. JEL classifications: E10; E40; E44; E47; E52; E60 Keywords: Potential output; output gap; financial cycle. Introduction Potential output has always been at the central point of economic policy making. Many policy decisions are conditional on the level of the potential output and output gap, the latter corresponding to the deviation of the potential from the actual level of output. Policymakers consider potential output as a key benchmark and use output gap as an input to adjust policy stance to reduce possible macroeconomic imbalances and control aggregate fluctuations. Potential output has been traditionally defined as the level of output compatible with stable inflation (Okun, 1962; Mishkin, 2007). The basic idea is that, ceteris paribas, inflation tends to rise when output is above its potential and vice versa. Alternatively, potential output has also been defined as the level that an economy can sustainably produce over the medium term in the absence of imbalances (Berger et al., 2015). These two definitions collectively suggest that traditionally inflation has been considered as the only key source of instability. Potential output is not directly observable; some combinations of economic and statistical reasoning must be used to estimate it from observed data. The estimate and the methodology used for estimation have been subjected to extensive analysis and scrutiny in the theoretical and empirical literature. This notwithstanding, the importance of this concept for economic policy will depend mostly on two factors. Firstly, as potential output measures the sustainable level of the output, the ability of output gap to reveal the imbalance of the economy is crucial. Secondly, the degree of uncertainty surrounding the estimates of the output gap and their robustness to new information determines the credibility of the estimate (Alberoa et al., 2013). Conceptually, the association between the output gap and inflation has been very strong and popular among the policy makers as it helps them to communicate their policy stance in the context of the short-run trade-off between output and inflation. As a result, traditional literature has largely ignored all factors, other than inflation, as a potential risk to sustainability. This premise has been questioned in recent period based on the global experience during the great moderation of an extended phase of low inflation coupled with expansion in economic activity, which was believed to be based on sound economic foundation and its eventual culmination in the form of global financial crisis in 2008. A closer look at the financial data reveals that imbalance in the sector was getting accumulated which eventually resulted in the crisis followed by a recessionary phase. This episode has clearly shown that gauging sustainability only in terms of price stability is too restrictive and may produce a misleading picture for policy makers, especially in a period of boom and bust. Also from the measurement perspective, it is widely accepted that financial development and cycle are closely related to the cyclical component of the output2. Bernanke, et al., (1999) in their “Financial Accelerator” model have argued that developments in credit markets amplify and propagate shocks to the macro economy. Stephane Dees (2016) is the latest work in this area where he shows that the importance of credit and asset price variables in explaining real economic fluctuations is relatively large. Hence, the output gap estimated ignoring financial sector information and using only inflation data would turn out to be less accurate, especially, when this is captured by cyclical component of the output. Recent research has started focusing on this deficiency. Studies in this area have concentrated either specifically on financial imbalances (Borio et al., 2013) or more broadly on macroeconomic imbalances (Alberoa et al., 2013). Table 1 displays the popularity gained by this methodology, especially among the central banking community. These measures could be more appropriately called sustainable output, rather than potential output, as the latter has been traditionally used in inflation / output trade-off sense. Generally, these types of imbalances tend to suggest that there exists a risk of significant output loss for a period of time. The recent financial crisis (2008), which was preceded by a strong credit growth, is an example of the ultimate result of such imbalance. Table 1: Recent Studies on Finance-Neutral Estimates of Output Gap | Authors | Published in | For Countries | Variables | Borio, Disyatat and Juselius (2013) | BIS Working Papers No 404 | US, UK and Spain | Credit and residen-tial property prices | Alberola, Estrada and Santab (2013) | Banco de Espa˜na Working Paper No. 1313 | US, UK, Spain Germany and China | Saving, investment, property prices and debt | Melolinna and To´th (2016) | BoE Staff Working Paper No. 585 | UK | Financial Conditions Index | Felipe, Sotocinal and Bayudan-Dacuycuy (2015) | ADB Economics Working Paper Series No.457 | G-5 countries and 10 high- and middle income Asian economies including India | Credit and property prices | Berger, Dowling, Lanau, Lian, Mrkaic, Rabanal and Taheri Sanjani (2015) | IMF Working Paper No. 15/233 | Euro Area | Credit and property prices | Alberola, Gondo, Lombardi, and Urbina (2016) | BIS Working Paper No. 568 | Latin American Countries | Commodity prices and capital inflows | ECB Bulletin - Box (2016) | ECB Bulletin 3/2016 | EMEs including India | Credit and capital flows | The moot question is whether one should consider rapid credit growth to be completely unsustainable. There exists strong argument in literature suggesting that rapid growth in financial sector is unsustainable with unusually strong financial booms likely to be coinciding with positive supply side shocks (Borio et al., 2013; Drehmann et al., 2012). Supply shocks exert downward pressure on prices while at the same time providing congenial environment for asset price booms that weaken financing constraints. This could lead to strong growth in the financial sector, if supported by monetary policy focused on stabilising near-term inflation (Borio and Lowe, 2002a). Secondly, these types of strong economic growth may themselves weaken the supply constraints. For example, prolonged and robust economic expansions can induce higher participation rate in labour market and thereby augmenting labour supply either by local or by immigrated labourers. By adding new capacity, the capital accumulation associated with economic expansion itself may also weaken supply constraints. Thirdly, the currency is expected to appreciate due to surge in capital flows as domestic assets become more attractive during the boom period, leading to downward pressure on inflation. Fourthly, unsustainability may have to do more with sector-wise misallocation of resources than with overall capacity constraints, with the sectors typically involved being those especially sensitive to credit, such as real estate. However, there exists another school of thought which believes this sort of credit expansion, at least a part of it, to be a result of sound policy and economic fundamentals. Considering the difficulty of identifying the drivers of a credit expansion in real time, it may not be wise to consider all such expansions as unsustainable; rather, these sorts of expansions need to be examined carefully, and may be treated as increasing the (downside) risks around a given baseline (Blagrave et al., 2015). Similar to global experience, India also witnessed high credit and asset price growth, and large positive output gap coupled with low inflation in the pre-crisis period3 (Prior to 2008Q3 in Figure 1). Crisis impact has manifested in large negative output gap for a year or so followed by a sharp recovery, with inflation gap getting into positive territory albeit showing high volatility initially. Credit and asset price gap remained choppy during this period. Subsequently, from 2014Q1 onwards, credit, inflation and output gaps moved into negative zone followed by the asset price. Although statistical measures like Hodrick-Prescott (HP) filter show positive output gap in 2015-16, the relationship between financial and business cycle is more or less evident from Figure 1. India-specific studies also have shown that credit and asset price channels of monetary policy transmission are gaining importance (Allen et al., 2011; Pandit and Vashisht, 2011; Khundrakpam, 2013). Peiris et al., (2010), by using a DSGE framework for India, shows that the extent of impact of a financial accelerator on output and inflation depends on the type of shock. Contractionary monetary policy shock induces more volatility in output and inflation compared to a technology shock. Khundrakpam and Jain (2012) found that credit slowdown and subdued asset prices have impacted GDP growth negatively. In view of this, the approaches which consider financial sector and broader macroeconomic imbalances, should be thought of as a complement to the traditional concept of potential output and not a substitute of it. 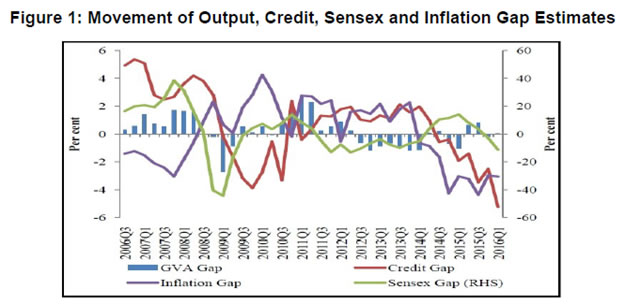 Borio et al., (2013) has showed that a substantial portion of the cyclical movements in output could be explained by the behaviour of credit and property prices proxying for financial cycle information. The measure of output gap, thus obtained, indicates high positive output gap during financial booms regardless of inflation. Similar exercises have been carried out by Alberola et al., (2013), Berger et al., (2015) and Melolinna and Tóth (2016) albeit for different set of countries and using somewhat different methodologies but arrive at similar conclusion. Although the above area is quite well-researched in the developed economies, there is a dearth of similar studies for emerging market economies, India in particular. Among the few studies available, Felipe et al., (2015) has examined the impact of financial factors on the output gap for the G-5 and 10 high- and middle-income economies in Asia including India4. Using real credit growth and real interest rate as financial sector variables, they have concluded that financial sector does not have significant impact on output gap. Very recently, Alberola et al., (2016) has estimated output gap measures for the Latin American countries by controlling for the commodity prices and net capital inflows. Focusing on India-centric studies, we find that interest in measuring the capacity output goes back to as early as 1970s (Divatia and Varma, 1970)5. Subsequently, many researchers have attempted to estimate potential output and the corresponding output gap for India (Donde and Saggar, 1999; Ranjan et al., 2007; Bordoloi et al., 2009; Mishra, 2013). Bhoi and Behera (2016) is the latest study on this topic where the authors have extensively applied different methodologies6 to estimate the potential output. However, all of them have focused on the potential output measure in the traditional sense. The recent episode of financial crisis has at least empirically validated that in the event of financial booms and busts, the real economy may proceed on an unsustainable path even if inflation remains low and stable. Thus, it may be useful to consider the financial variables while making judgment on the sustainable level of economic activity and assess the state of the economy through the lens of a “finance-neutral” measure of potential output. The primary objective of this paper is to fill this gap by capturing the information content in financial variables for the cyclical part of the output and filter it out to estimate the potential level for the Indian economy. The rest of the paper is organised as follows. In Section II, we present the framework and the methodology used for our estimation strategy which includes financial variables in the IS curve specification. In Section III, we discuss the data used in paper and present the results. Section IV concludes the study. II. Estimation of Potential Output and Output Gap with Financial Variables Univariate statistical filters, such as the HP filter, are widely used to estimate potential output and output gap. This approach can be applied directly to the GDP series without taking into account any information on other variables. In that sense it is simple and convenient, especially, in the context of data scarcity and hence much suited for emerging market economies. However, the simplicity of this approach brings with it several limitations. The major criticism against statistical filters is that they do not have any economic concept or underlying economic dynamics, being merely a statistical approach. Another popular approach for estimating potential output is using production function, in which the inputs of production - capital and labour - are considered separately. The advantage of this approach is that it allows explanations of the drivers of potential output. However, there are limitations, particularly, specific to emerging economies like India which have data constraints like timely availability of capital-stock and nation-wide employment data in a regular frequency. In this context, possibly, the best suited approach is to use semi-structural models like multivariate filters to estimate potential output (Laxton and Tetlow, 1992; Kuttner, 1994; Benes et al., 2010; Blagrave, 2015). On one hand this approach is consistent with Okun’s concept of potential; on the other hand in its simplest form, this approach is easier to put into practice as it requires only few variables. Recently, a competing literature has evolved which utilizes financial sector information for estimating output gap and potential output (Borio et al., 2013). In this paper, we make use of that approach to estimate potential output and output gap7 which are considered to be much more robust in real time. 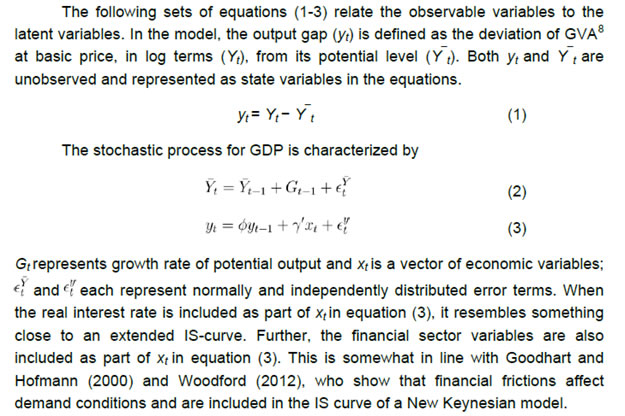 Accordingly, the set of equations (1) to (3) represents an approximation of economic theory and statistical filtration in estimating potential output and output gap, incorporating financial sector developments.9 We consider different specifications of (3), but primarily focus on three different financial variables: a) bank credit, b) asset prices and c) financial conditions index (FCI). Some recent papers have used FCI to represent the composite behavior of financial cycles while estimating finance neutral output gap (Melolinna and Tóth, 2016). In our paper we have used a measure of FCI developed by Shankar (2014)10 for India. The aggregate FCI for India synthesizes the information from money, bond, exchange rate and equity markets. However, this index does not cover the credit markets. Hence in the regressions carried out with FCI as an explanatory variable, credit market variable is also included along with FCI at suitable lags. Further we allow each of these variables to enter (3) only once at a suitable lag so as to maximise the statistical fit. In state-space models, observed dependent variables are written down as functions of unobserved state variables and allow both the observed dependent variables and the unobserved state variables to be functions of exogenous variables. The system of equation is estimated using state space models by Kalman Filter. Specifically, as the model is non-stationary, diffuse Kalman filter (De Jong, 1988, 1991) is used. The parameters are estimated using Quasi maximum likelihood (QML) method. III. Data and Results The empirical analysis starts with identifying the appropriate variables representing measurement of different variables used in the specification (1) to (3). We have used the Gross Value Added (GVA) series at base 2011-12 for measuring output (Yt). As the back series of GVA is not officially available, data for earlier periods were obtained by splicing. This series is seasonally adjusted and represented in natural logarithm. Real policy rate (it) is represented by the repo rate deflated with the one period ahead inflation. Bank credit is measured using the quarterly growth rate in the seasonally adjusted non-food credit. In the model, real bank credit (bct) is used. This is obtained by deflating the bank credit using CPI. Similar treatment is used for BSE sensex (sensext), which is used to represent the asset price. All observed variables are de-meaned. Analysis is carried out for the period Q1:2006-07 to Q4:2015-16. All data have been sourced from Database on India Economy, RBI (https://www.rbi.org.in). The benchmark for the choice of suitable lags for each of the variables is based on univariate analysis, cross correlations and OLS regression between output gap and variables mentioned above11. Policy rate affects output gap with a lag of 3-6 quarters. Bank credit seems to affect output gap with a lag of 2 quarters. Assets prices affect demand conditions in 1-2 quarters. Equations (1) to (3) are estimated using five different model specifications. No financial sector variable is used in Model 1. Model 2 uses it and bct as the determinants of output gap. it and sensext are included in xt in Model 3. Model 4 uses credit and asset variables in the IS equation along with real policy rate. Thus, we have xt= (it−h, bct−h, sensext−h) and γ = (γ1,γ2,γ3). Model 5 estimates regression with FCI as one of the explanatory variables along with real credit growth and real policy rate. The results are presented in Table 2. Table 2: Regression Estimates of State-Space Models IS Curve (Output gap equation) | Parameter | Model 1 | Model 2 | Model 3 | Model 4 | Model 5 | φ | 0.53 (0.01) | 0.56 (0.00) | 0.48 (0.00) | 0.44 (0.00) | 0.18 (0.35) | γ1 | -0.16 (0.09) | -0.16 (0.03) | -0.18 (0.04) | -0.19 (0.01) | -0.23 (0.01) | γ2 | – | 0.09 (0.21) | – | 0.14 (0.01) | 0.11 (0.05) | γ3 | – | – | 0.04 (0.00) | 0.04 (0.00) | -0.33* (0.00) | Note: The models are not stationary. Estimated using QML method; p-values are given in brackets. * Estimated with FCI in place of sensex. Smaller values of FCI represent favorable financial conditions. Therefore lower values of FCI is expected to contribute to uptick in economic cycles and vice versa, hence the negative coefficient value (γ3). | As can be seen from Table 2, real policy rates do affect output gap significantly with a negative sign as expected. For all the regression equations the chosen lag12 for the policy variable is 4. The real credit affects demand significantly in 2 quarters. Real return of asset prices is also significantly related to output gap with expected sign. The regression result of Model 5 suggests that FCI significantly affects output gap with a lag of one quarter. The finance neutral estimates of output gap and potential output have been estimated using Model 4. The estimates of the potential output and output gap from these specifications along with a HP filtered one are presented in the Figure 2 (a-c) for comparative purposes. 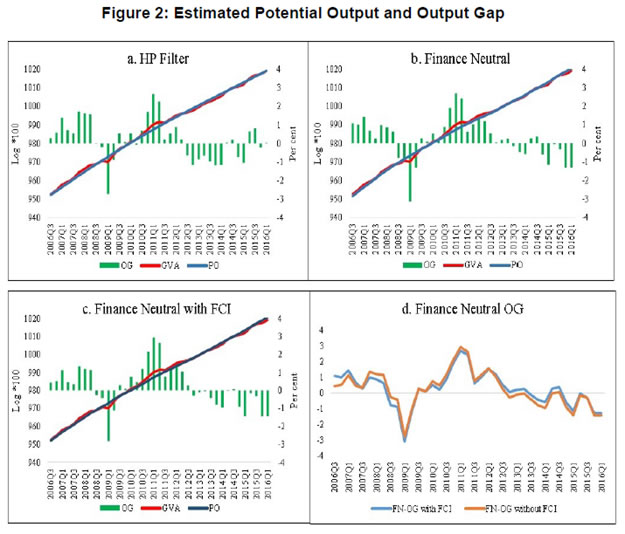 The pre-crisis period (prior to 2008) showed a positive output gap underscoring an excess demand scenario in the economy. During the global financial crisis demand collapsed but recovered quite sharply. Subsequently, a couple of years (2010-2012) witnessed an excess demand scenario and the economy witnessed prolonged period of negative output gap since then. The HP filter shows that the output gap has almost closed in recent quarters while the finance neutral output gap indicates that there exists considerable slack in the economy. The estimated output gaps from Model 4 and Model 5 are broadly similar suggesting the robustness of estimated finance neutral output gap measure (Figure 2(d)). 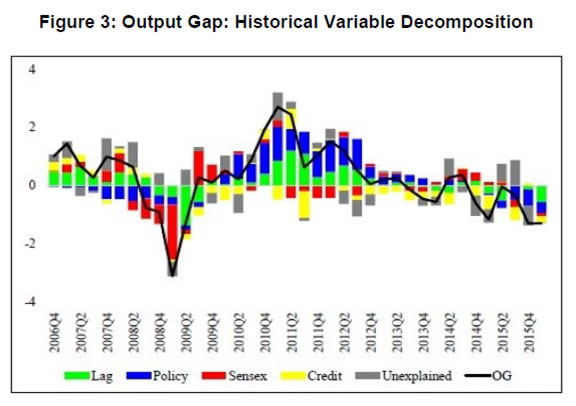 The historical variable decomposition of output gap, estimated using Model 4, portraying the contribution of various factors on its evolution has been presented in Figure 3. The pre-crisis period witnessed positive contribution of financial variables to demand conditions. During this period, real policy rate was playing a dampening role on demand conditions. The global financial crisis flipped the role of financial variables and started to contribute negatively to output gap. High inflation and negative real interest rates during the period 2010-13 contributed positively to output gap. More recently the subdued credit growth and real policy rate have supposedly contributed negatively to demand conditions. IV. Conclusion The estimation of output gap incorporating financial factors is gaining wide popularity, particularly, among central bankers. The burgeoning literature in this sphere has predominantly covered the developed economy context in the recent period stressing the importance of the financial variables in explaining the cyclical component of economic activity and hence potential output. Notwithstanding the period of benign inflation and high output growth, the extreme movements in financial variables were often found unsustainable and mostly contributed to imbalances even in the emerging market economies, particularly in the pre-crisis period. Following this strand of literature, this paper is an empirical attempt to examine whether a similar relationship exists in an emerging market economy like India and estimate output gap and potential output applying a multivariate Kalman filter using macro and financial sector variables. The results presented in our paper suggest that asset price and credit market movements are seen to affect demand conditions with a lag. Similar result is found when a financial conditions index is used in place of the asset market indicator, suggesting the robustness of the above result. Our estimate of finance-neutral output gap may be considered as a useful addition to the policy maker's toolkit by providing a different perspective on the state of economic cycle in India. References Aikman, D., A. G. Haldane and B. D. Nelson (2011). “Curbing the Credit Cycle,”Speech presented at the Columbia University Center on Capitalism and Society Annual Conference, New York. Alberola E, A. Estrada and D. Santabrbara (2013). “Growth beyond Imbalances. Sustainable Growth Rates and Output Gap Reassessment”, Banco de Espana Working paper, No.1313. Madrid. Alberola, E., R. Gondo, M. J. Lombardi and D. Urbina (2016). “Output gaps and policy stabilisation in Latin America: the effect of commodity and capital flow cycles,” BIS Working Papers, No. 568, June. Alessi, L. and C. Detken (2009). “Real Time’ Early Warning Indicators for Costly Asset Price Boom/Bust Cycles: a Role for Global Liquidity,” ECB Working Paper, No. 1039, Allen, F. and K. Rogoff (2011). “Asset Prices, Financial Stability and Monetary Policy,” Swedish Riksbank Workshop on Housing Markets, Monetary Policy and Financial Stability, Baxter, M. and R. G. King (1999). “Measuring Business Cycles: Approximate Band-Pass Filters For Economic Time Series”, Review of Economics and Statistics. Beneš, J., K. Clinton, R. García-Saltos, M. Johnson, D. Laxton, P. B. Manchev and T. Matheson (2010). “Estimating Potential Output with a Multivariate Filter,” IMF Working Paper, WP/10/285. Bernanke, B. S., M. Gertler and S. Gilchrist (1999). “The financial accelerator in a quantitative business cycle framework”, Handbook of macroeconomics, 1 (1999): 1341-1393. Bhoi, B.K. and H. Behera (2016). “India’s Potential Output Revisited”, RBI Working Paper Series, WPS (DEPR): 05 / 2016. Bordoloi, S., A. Das and R. Jangili (2009). “Estimation of Potential Output in India”, Reserve Bank of India Occasional Papers, 30(2): 37-73. Borio, C., P. Disyatat and M. Juselius (2013). “Rethinking Potential Output: Embedding Information about the Financial Cycle,” BIS Working Papers, 404, February. Borio, C. E. and M. Drehmann (2009). “Towards an Operational Framework for Financial Stability: “Fuzzy” Measurement and its Consequences”, BIS Working Papers, No. 284, June. Borio, C. E. and P. W. Lowe (2002). “Asset Prices, Financial and Monetary Stability: Exploring the Nexus,” BIS Working Paper, No. 114, July. Berger, H., T. Dowling, S. Lanau, W. Lian, M. Mrkaic, P. Rabanal, and M. Taheri Sanjani (2015). “Steady as She Goes Estimating Potential Output During Financial Booms and Busts,” IMF Working paper, WP/15/233, November. Blagrave, P., M. R. Garcia-Saltos, M. D. Laxton and F. Zhang (2015). “A Simple Multivariate Filter for Estimating Potential Output,” IMF Working Paper,,No. WP/15/79, April. Claessens, S., M. A. Kose and M. E. Terrones (2011 a). “Financial Cycles: What? How? When?,” IMF Working Paper, WP/11/76, April. ————–(2001 b). “How Do Business and Financial Cycles Interact?,” IMF Working Paper, WP/11/88, April, 2011. Christiano, L. J. and T. J. Fitzgerald (2003). “The Band Pass Filter,” International Economic Review, Volume 44, Issue 2, 435465, May. Dees, S. (2016). “Credit, Asset Prices and Business Cycles at the Global Level,” ECB Working Paper, No 1895, April. De Jong, P. (1988). “The Likelihood for a State-Space Model,” Biometrika, 75, 165169. ————–(1991). “The Diffuse Kalman Filter,” The Annals of Statistics, Vol 19, No. 2, 1073-1083. Divatia, V.V. and R. Varma (1970). “Index of Potential Production and Potential Utilisation Ratio for the Manufacturing Industries in India,” Reserve Bank of India Bulletin, April. Donde, K. and M. Saggar (1999). “Potential Output and Output Gap: A Review.”, Reserve Bank of India Occasional Papers, 20(3): 439-467. Drehmann, M., C. E. Borio, and K. Tsatsaronis (2012). “Characterising the Financial Cycle: Don’t Lose Sight of the Medium-term!,” BIS Working Papers, No. 380. Felipe, J., N. R. Sotocinal and C. Bayudan-Dacuycuy (2015). “The Impact of Financial Factors on the Output Gap and Estimates of Potential Output Growth,” ADB Economics, Working Paper Series, No. 457, October. Goodhart, C., and B. Hofmann (2000). “Asset prices and the conduct of monetary policy,” Sveriges Riksbank and Stockholm School of Economics conference on Asset Markets and Monetary Policy, Stockholm, June. Hodrick, R. J., and E. C. Prescott (1997). “Postwar U.S. Business Cycles: An Empirical Investigation,” Journal of Money, Credit and Banking, Blackwell Publishing, vol. 29(1), pages 1-16, February. Kalman, R. E. (1960). “Credit Channel of Monetary Policy Transmission in India: How Effective and Long is the Lag?,”, Journal of Basic Engineering, 82 (1): 3545. doi:10.1115/1.3662552. Khundrakpam, J. K. (2013). “Credit Channel of Monetary Policy Transmission in India: How Effective and Long is the Lag?,” The IUP Journal of Applied Economics, Vol. 12(1), 2649. Khundrakpam, J. K. and R. Jain (2012). “Monetary Policy Transmission in India: A Peep Inside the Black Box,” RBI Working Paper Series (DEPR), 11/2012. Kuttner K.N. (1994). “Estimating Potential Output as a Latent Variable,” Journal of Business and Economic Statistics, Vol. 12, 36168. Laxton, D., and R. Tetlow (1992). “A Simple Multivariate Filter for the Measurement of Potential Output,” Technical Report, Ottawa: Bank of Canada, No. 59. Okun, A.M. (1962). “Potential GNP: Its Measurement and Significance,” In Proceedings of the Business and Economic Statistics Section,(Washington: American Statistical Association)., pp. 98-104. Melolinna, M., and M. Tóth (2016). “Output Gaps, Inflation and Financial Cycles in the United Kingdom,” Bank of England, Staff Working Paper, No. 585, February. Mishkin, F.S. (2007). “Inflation Dynamics,” Annual Macro Conference, Federal Reserve Bank of San Francisco, March. http://www.federalreserve.gov/newsevents/speech/Mishkin20070323a.htm. Mishra, P. (2013). “Has India’s Growth Story Withered?,” Economic and Political Weekly, 48(15): 51-59. Pandit, B. L. and P. Vashisht, (2011). “Monetary policy and credit demand in India and some EMEs,” Indian Council for Research on International Economic Relations, Working Paper, No. 256. Peiris, S. J., M. Saxegaard and R. Anand, (2010). An estimated model with macro-financial linkages for India. IMF Working Papers, 1-44. Ranjan, R., R. Jain and S.C. Dhal, (2007). “India’s Potential Economic Growth: Measurement Issues and Policy Implications,” Economic and Political Weekly, 42(17): 1563-72, 2007. Jordà, Ò, M. H. Schularick, , and A. M. Taylor (2011). “When Credit Bites Back: Leverage, Business Cycles, and Crises,” Federal Reserve Bank of San Francisco Working Paper,, 2011-27. Shankar, A. (2014). A Financial Conditions Index for India, Reserve Bank of India Working Paper Series, W P S (DEPR): 08 / 2014. Woodford, M (2012). “Inflation Targeting and Financial Stability,” NBER Working Paper, No 17967. |